Predicting intentional accounting misreporting
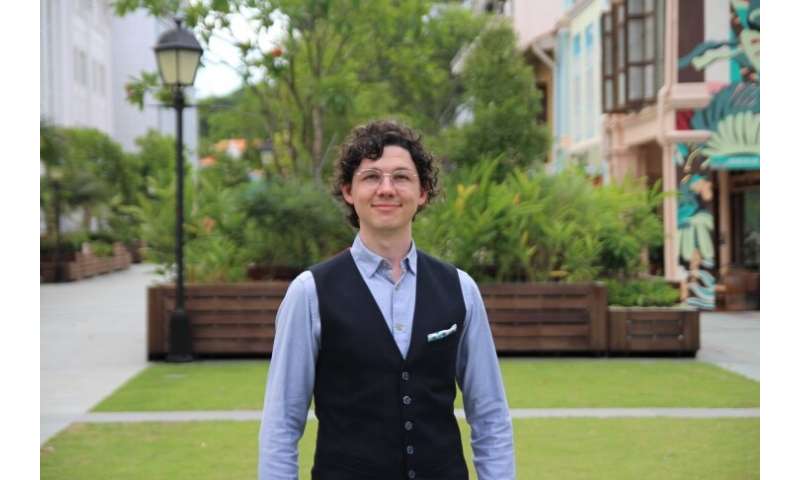
In the U.S. Securities and Exchange Commission (SEC) 10-K annual report filing for its financial year ending July 31, 2008, American jewellery retailer Zale Corporation ('Zales') mentioned the words 'advertising' or 'advertisement' 17 times. A year later, those same words showed up more than twice as often at 41 times.
By then, the SEC had begun investigations after the company delayed posting fourth-quarter results. Zales was subsequently found to have improperly capitalised television advertising costs from 2004 to 2009 although few had noticed what was going on.
In a method featured in new research by SMU Assistant Professor of Accounting Richard Crowley, this intentional misreporting would have sent alarm bells ringing well before the SEC started asking questions.
"They're 97th percentile or higher in our model in every single year from the second year of misreporting onwards," says Professor Crowley, referring to the machine learning technique featured in the paper "What are You Saying? Using Topic to Detect Financial Misreporting". "97th percentile here means that their score on our misreporting detection model was higher than 97 percent of U.S. public companies."
He adds: "The model is run yearly, so that means that for each year of 2005, 2006, ... 2009, Zales scored a higher misreporting detection score than 97 percent of public companies that year."
What's the word?
Professor Crowley explains that the research completely ignores the numbers—"If managers are going to misreport the numbers, they're going to do it in a believable fashion"—and instead looks at what is written instead, which the research refers to as the 'topic'.
Together with Professors Nerissa Brown and Brooke Elliott from Gies College of Business at the University of Illinois Urbana-Champaign, Professor Crowley analysed over 3 billion words in 10-K filings from 1994-2012 to see how reliably certain topics predicted intentional misreporting. In certain samples, the research improved prediction of intentional misreporting by 59 percent.
"The one key difference when you're discussing things when you're lying is that you're very intentional on the topics you pick to discuss," he elaborates, pointing to the example of Enron.
"They just talk about increases in income and they have an enormous amount of discussion about that," Professor Crowley observes. Enron's 1999 annual report serves as a prime example, citing "acceleration of Enron's staggering pace of commercial innovation" for a 28 percent revenue increase to US$40 billion from a year ago, as well as a 37 percent jump in net income before non-recurring items to US$957 million.
Professor Crowley singles out a phrase that Enron used often in their 10-K's: "compared with". He explains:
"Companies are always saying things like, 'This is our income in 2011 compared with income in 2010,' and they're always giving forecasts about income, gross margins etc.
"But then you have income taxes, non-interest income, profit, those are just the general phrases that show up. When we picked out the most representative sentences for each of these topics, we found phrases such as 'operating profit was $122.1 million in 2011 compared with $113.9 million to 2010, an increase of 7.8 percent.' This is an extremely common structure to see in these documents.
"So when we talk about Enron, they have sentences like that, but they have a lot more of them than anybody else has ever done, both in 1999 and across the entire history of our sample."
Given the purported number of deals Enron had that generated all that revenue, it might make more sense to read in its annual reports things such as acquiring sources for its energy contracts, Professor Crowley notes. Instead, it largely "talked about revenue figures and income figures", he observes.
So is there a tipping point of the number of times a topic appears that is a red flag? Or the kinds of words used?
"There is no constant sort of barometer for this," Professor Crowley tells the Office of Research and Tech Transfer. "I can't just say if they talked about it X percent of the time, we got them. It depends on a lot of factors. And a lot of these factors are industry-specific, and some are firm-specific.
"[It also depends on whether] you're in a recession versus if you're not in a recession. Likewise, if you're a financial company versus a healthcare company, or a phone company versus a steel manufacturer, [the topics to look for] should all be different."
You can't game what you don't know
Professor Crowley and his collaborators employed over 20 different text-based variables in their predictive model, including the use of the Fog Index for readability.
While intuition would suggest an easy to read 10-K to be transparent, Professor Crowley counters by saying "it could be because they left out all the details". Similarly, positive sentiments like those expressed by Enron could be signals of intentional misreporting, although it is impossible to be 100 percent sure.
"It takes just six seconds to run through a 10-K with our model," Professor Crowley says while noting that the SEC has adopted parts of his model to uncover intentional misreporting. But the question must be asked: Can firms looking to mislead the market study the algorithm to beat the SEC at its own game?
"The one nice thing about this algorithm is that it changes every year," he elaborates, pointing to the combination of words that make up the topics that the algorithm works on. "Companies don't know what the regulator's target would be, even if they're using our algorithm."
"The benefit of that is that if you're a company trying to manipulate, you don't know what the target is either."
More information: Nerissa C. Brown et al. What are You Saying? Using Topic to Detect Financial Misreporting, SSRN Electronic Journal (2016). DOI: 10.2139/ssrn.2803733
No comments:
Post a Comment