BERNIE TOVARICH BOTS

March 11, 2020 By Keith A. Spencer, Salon- Commentary

Mainstream pundits and politicians continue to obsess over the stereotype of the “Bernie Bro,” a perfer vid horde of Bernie Sanders supporters who supposedly stop at nothing to harass his opponents online. Elizabeth Warren, Hillary Clinton and New York Times columnist Bret Stephens have all helped perpetuate the idea that Sanders’ supporters are somehow uniquely cruel, despite Sanders’ platform and policy proposal being the most humane of all the candidates.
The only problem? The evidence that Sanders supporters are uniquely cruel online, compared to any other candidates’ supporters, is scant; much of the discourse around Bernie Bros seems to rely on skewed anecdotes that don’t stand up to scrutiny. Many Sanders supporters suspect that the stereotype is perpetuated in bad faith to help torpedo his candidacy.
A few weeks ago I penned a story for Salon attempting to qualitatively disprove the Bernie Bro myth by pulling from psychological theory and the nature of online behavior. To summarize my conclusions: First, there is a general tendency for online behavior to be negative, known as the online disinhibition effect — but it affects all people equally, not merely Sanders’ supporters. Second, pundits systematically ignore when other candidates’ supporters are mean online, perhaps because of the aforementioned established stereotype; in this sense, the Bernie Bro is not dissimilar from other political canards like the “welfare queen.” Third, Twitter is not a representative sample size of the population, and is so prone to harboring propaganda outfits and bots such that it is not a reliable way of gauging public opinion.
Now, to add to this qualitative assessment, there is quantitative evidence, too — reaped from studying hundreds of thousands of interactions online — that reveals the Bernie Bro myth as, well, a myth. Jeff Winchell, a computational social scientist and graduate student at Harvard University, crunched the numbers on tweet data and found that Sanders’ supporters online behave the same as everyone else. Winchell used what is called a sentiment analysis, a technique used both in the digital humanities and in e-commerce, to gauge emotional intent from social media data.
“Bernie followers act pretty much the same on Twitter as any other follower,” Winchell says of his results. “There is one key difference that Twitter users and media don’t seem to be aware of…. Bernie has a lot more Twitter followers than Twitter followers of other Democrat’s campaigns,” he added, noting that this may be partly what helps perpetuate the myth.
I interviewed him about his work and his results over email; as usual, this interview has been condensed and edited for print.
First, for those who haven’t heard of this technique, what is a sentiment analysis?
Sentiment analysis summarizes human expression into various scores. Most commonly the score is how negative or positive it is. But it can also be used to evaluate subjectivity (for instance, is a politician’s statement factual or mostly opinionated?). Even taking the simpler text analysis, there are multiple challenges due to sarcasm, negations (e.g “I don’t like their service”, “After what he did, this will be his last project”), ambiguity (words that are negative or positive depending on their context), and [the fact that] texts can contain both positive and negative parts.
How are sentiment analyses used? What are other examples of this technique being used?
The overwhelming application of sentiment analysis is in e-commerce (for instance, scoring how positive/negative customer feedback is). Customer service surveys are often analyzed this way. Marketing uses sentiment analysis to test product acceptance.
Other commercial applications are in recommendations. While a system may have the user given an overall rating, analyzing the comments they provide can identify the sentiment on subtopics within.
So tell me about the sentiment analysis script that you wrote to study online behavior among different politicians’ followers. How did this work?
I downloaded all the followers of the Twitter accounts of the nine most popular Democratic presidential candidates and the president ([around] 100 million Twitter accounts). I then randomly chose followers from them and downloaded all their tweets from 2015 to the present.
I have run two different sentiment analysis algorithms on these tweets. So far, nearly 6.8 million tweets from 280,000 Twitter accounts have been analyzed out of the 100 million-plus tweets I currently have downloaded (I continue downloading more).
One sentiment analysis algorithm uses a well-regarded example of grammar/word dictionary sentiment rules that were popular 5 to 10 years ago before deep learning became popular. This one is identified by the Python libary’s name, Textblob.
The other algorithm is Microsoft’s supervised deep learning-based algorithm with default parameters. To those unfamiliar with deep learning, the number of parameters in this model is in the millions, and no human can be expected to understand them. The deep learning model learns/generalizes from examples of text given sentiment ratings by humans through millions of trials, each time evaluating how well it predicts the results and passing that model and accuracy to the next iteration.
The categories of negative and very negative are based on ranges of values in the two algorithm’s outputs. Textblob generates a number from most negative (-1) to most positive (+1). I classified scores of [below] -0.75 as very negative and -.75 to -.5 as negative. Microsoft’s algorithm predicts the chance that some text is classified as positive. Based on the frequencies of a specific chance, I separated the lowest 1.5 percent of tweet ratings as very negative and the lowest 1.5 percent to 5 percent of all tweet ratings as negative.
What did your results find?
The chance that some tweet is negative when it comes from a follower of candidate X is pretty much the same as if it came from a follower of candidate Y.
This uses two different algorithms, once very sophisticated (Microsoft’s supervised Deep Learning-based model), the other a good algorithm based on the algorithm standards of 5 to 10 years ago (Textblob’s grammar/dictionary-based rules). Microsoft’s algorithm calculates the chance a tweet is positive. Textblob’s rates the tweet from most negative (-1) to most positive (+1). But the variation of these measures changes little among tweets from followers from different candidates.
I deliberately round my numbers to 1 digit for smaller samples (negative or very negative percentage) or 2 digits if it’s about an average over all the tweets. I don’t like false accuracy and it is rampant in the political media. Any NLP [Natural Language Processing] expert will tell you that reducing a tweet to a single number denoting its negativity/positivity is not an exact science. So the rounding reflects that uncertainty.
Given this data, what do you think of the “Bernie Bro” narrative about his online supporters?
Bernie followers act pretty much the same on Twitter as any other follower. There is one key difference that Twitter users and media don’t seem to be aware of. Bernie has a lot more Twitter followers than Twitter followers of other Democrat’s campaigns.
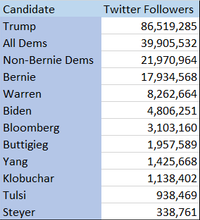
People responding to hundreds of millions of people online tend to dehumanize others. They remember that someone is female/male or follows some candidate or is of some race, but they frequently don’t pay attention to differentiate actions of one member of that group versus another. So rather than consider how frequently an individual of some group acts, they think of how frequently the group acts as a whole. If they interact with many more members of one group than another, that perception of the group is magnified by the number of members they see.
Interesting. Did your opinion change after doing this little analysis?
Yes. I believed that Bernie’s followers are more likely to like him because they are more likely to experience the very negative life circumstances that Bernie Sanders wants to fix. People in a negative situation are more likely to interact negatively with people, particularly those anonymous online people that they have no in-person relationship with. So I had anticipated that Bernie’s followers on average would have a much higher chance to be negative. This does not appear to be the case or at least not as much as the claims I read on Twitter, political media reports or on TV.
Is there actually any difference between different candidates’ supporters online behavior, based on this?
As a data scientist, I am usually skeptical of any result. So I’ll say maybe not or at least much less than claimed.
I still would like to dig deeper into this. This analysis looks at all tweets. I would like to look just at twitter interactions between candidate’s supporters, look at tweets responding or mentioning media professionals. I want to use some algorithms in the research that evaluate hate speech, racism, sexism. I’d like to look at specific topics of discussion, and possibly evaluate the influence of negative tweets (eg. retweets and number of followers who could see a tweet/retweet).
What is your academic background?
I have a bachelor’s degree in math from Northwestern. I then worked in healthcare analytics with very large databases, branched into other applications of large scale data analysis before recently returning to grad school at Harvard to study data science. While there my interest in psychology and sociology has led me to pursue applications of data science in the social sciences to help people.
This story was updated on March 10 with additional interview questions to add context.
No comments:
Post a Comment