Beyond the Curve: Dr. Peter Lurie's COVID-19 Blog
September 12, 2020
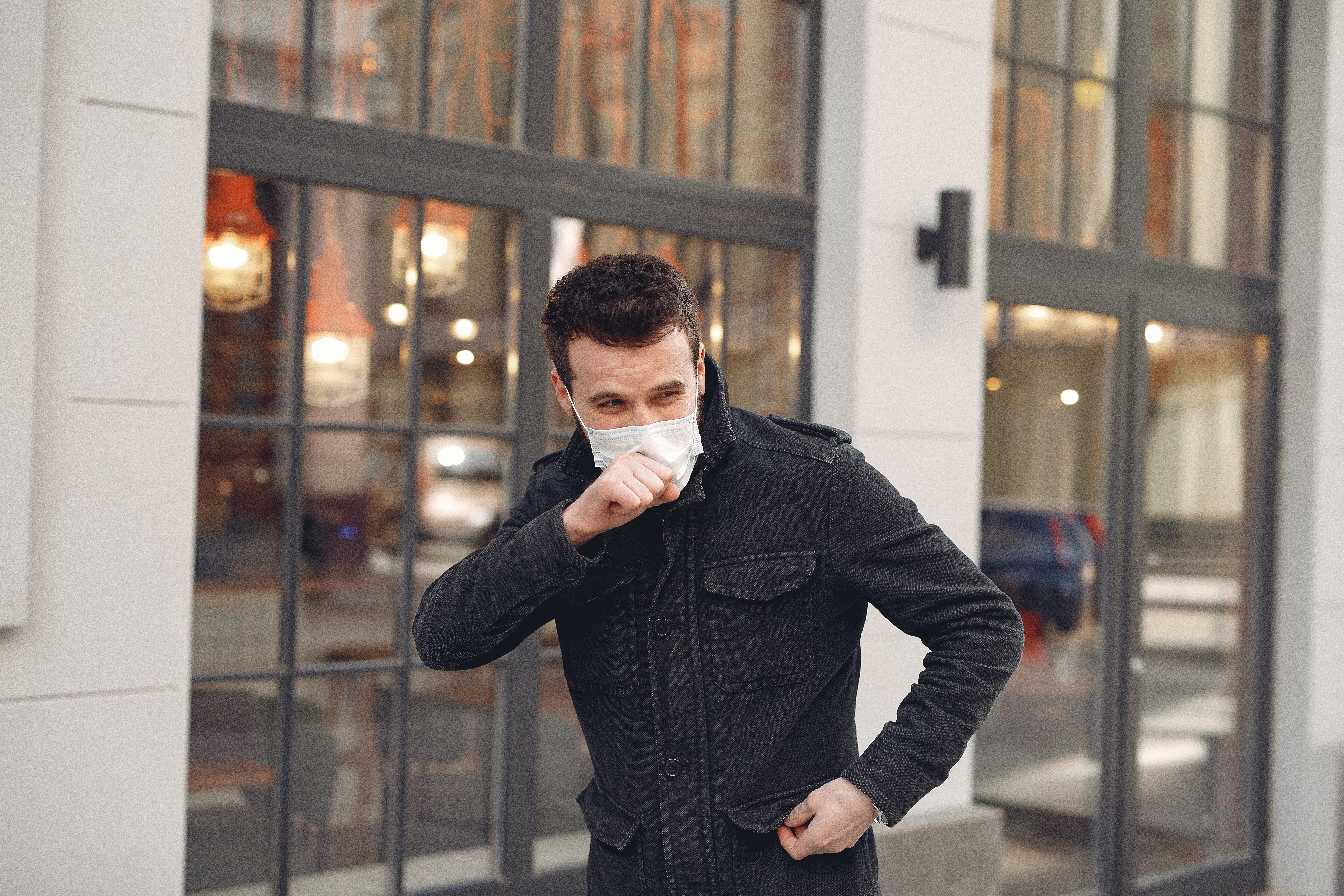
This is a guest post by CSPI senior policy associate Ashley Hickson. See all Beyond the Curve posts.
A salutary benefit of the coronavirus pandemic is how it underscores that we’re all in this sticky predicament together. In order to “flatten the curve,” we all have to act in concert by wearing masks, washing hands, respecting social distancing recommendations, and generally minimizing contact with others. In epidemiology, this notion of interdependence is encapsulated in the concept of “herd immunity.” When most of a population is immune to an infectious disease, this provides indirect protection—or herd immunity—even to those who are not immune to the disease. In essence, once population immunity reaches a certain level, your chances of encountering someone who is infectious decline significantly. Depending on how infectious a disease is, usually 70% to 90% of a population needs to be immune for herd immunity to be established. But antibody levels, which measure prior exposure to the virus, are only around 25% in New York City, and considerably lower almost everywhere else. And this assumes that having antibodies assures immunity – something that has not yet been proved. Thus, the majority of the population remains susceptible to infection.
Given the high proportion of still-susceptible individuals, it’s unsurprising that COVID-19 outbreaks are persisting in cities such as Chicago, Houston, Los Angeles, Miami, and Phoenix. These dismal events paint a picture of an outbreak that is not contained and is still spreading rapidly.
How do epidemiologists portray the potential for spread of an infectious disease? Here, we turn to a technical term you may have heard about (and certainly will): the basic reproduction number, denoted as R0 (pronounced "R naught"). This measure describes the likely impact of a single infection—the number of people to whom an infected person will likely transmit the disease if no one is immune. It is one of the most fundamental metrics in the study of infectious disease outbreaks. So, if each person spreads the infection to two people and each cycle of infection takes a week, after about a month 16 people have been infected (of course, in that period, some of the 16 will have recovered).
To understand, from a theoretical standpoint, how R0 is calculated, consider a single infected person who contracts a disease at the beginning of an outbreak when no one is immune. To calculate how many people that person will infect, we must first figure out how long the person is infectious (2 to 14 days for COVID-19, according to the Centers for Disease Control and Prevention) and multiply that by the number of people they encounter per day. The number of contacts encountered can be calculated either at the individual- (via contact tracing) or population-level. Finally, we multiply that by the likelihood of infection for each encounter because, thankfully, the great majority of encounters do not lead to infection. That gives us the number of people who were infected by the first person. Presto! It’s R0 itself. (In practice, R0 is calculated using complex mathematical modeling with several additional inputs, but this illustrates the concepts behind the measure).
To help contextualize R0, the 1918 Spanish Influenza pandemic that killed 50 million people worldwide had an R0 that was estimated at between 1.4 and 2.8. The seasonal flu is estimated to have a R0 of approximately 1.3. Although estimates of R0 for COVID-19 vary, a range of 2 to 3 is often cited.
But, typically, shortly after an outbreak begins, some people become immune, so R can vary over time. This is where the effective reproductive number, or Rt, comes in (t stands for time). This number describes whether outbreaks are growing, remaining steady, or decreasing. An Rt value greater than 1 indicates that the epidemic is continuing to grow, an Rt value of 1 indicates that the outbreak is stable (with each case spreading the disease to one person), and an Rt less than 1 means the outbreak is on the wane and, all things being equal, will extinguish itself.
In addition to R0 and Rt, the dispersion factor, or “k” is also important in assessing an outbreak. K captures the idea that some people will spread the infection to many people, while others won’t spread it to anyone else, depending on social distancing and a host of other mitigating factors like mask wearing. For example, research is showing that people infected with COVID-19 are most infectious for a brief period of time; if an infected person enters a high-risk setting during this period, a super-spreading event could occur. Other interactions, even by the same individual, will confer lower risk. So k captures a level of complexity not contained in R0 or Rt: all exposures are not created equal. In general, the distribution of infectiousness depends upon the virus, characteristics of the potential spreader, and the circumstances in which infections are spread, including efforts to mitigate transmission.
We have examples of this domestically from outbreaks associated with small social events. These gatherings, such as bible study groups or birthday parties, are often more likely to take place indoors, creating an environment where the virus is able to spread easily from person to person. In Texas, a family hosted a birthday party for a total of 25 guests on May 30th. A single relative, unknowingly infected with the virus that causes COVID-19, spread the disease to seven family members (much greater than the typical value for R0); from this encounter, ten relatives who did not attend the party were infected. Across the nation, Greek fraternity and sorority houses, along with residence halls, have become the site of COVID-19 outbreaks at universities including Oklahoma State and the University of North Carolina at Chapel Hill. An outbreak at a sleep-away camp in Georgia last month resulted in 260 children and staffers contracting the virus less than a week after spending time together in close quarters. This example is the largest documented super-spreading event among children domestically, and gives pause for concern around the upcoming school year.
Unlike R0, the calculation for k is a bit too involved to discuss here. Suffice it to say, it captures the heterogeneity in likelihood of transmission in a given population. If a small number of individuals are responsible for a large number of transmissions, the value of k will be close to zero. As we continue to evaluate how this disease spreads, k will be a critical measure to consider when making decisions about reopening schools and businesses.
A research study modeling COVID-19 outbreaks across different countries discovered that k is around 0.1, implying that as few as 10% of infected individuals may be associated with 80% of transmissions. This provides a ray of hope: if we focus on potential super-spreading events like, ahem, indoor political rallies, we can have a disproportionate impact upon the course of the pandemic.

Ashley Hickson is a Senior Policy Associate focusing on healthy retail campaign initiatives. Prior to joining CSPI, she served as Director of Community Impact at the American Heart Association where she led her market’s food access strategies. Ashley is a Doctor of Public Health student at Johns Hopkins with a concentration in Health Equity and Social Justice. She has a passion for food justice and is a Bloomberg American Health Initiative Fellow in the obesity and food system focus area. She holds an MPH from the University of Texas Health Science Center
No comments:
Post a Comment