by Ng Yi-Di, Singapore Managment University
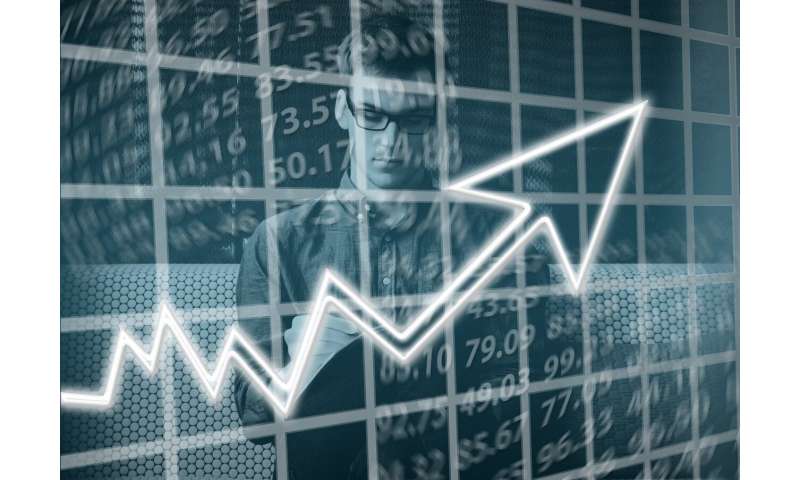
Credit: CC0 Public Domain
Danish physicist Neils Bohr once quipped that prediction is hard, especially when it is about the future. But this is precisely what financial regulators need to do—forecasting the likely state of the economy in the future is crucial when deciding on policy levers like whether to slash or raise interest rates.
However, as the world continues to become more unpredictable, forecasting has become increasingly difficult. This challenge was poignantly illustrated after the start of the 2008 Financial Crisis, when Queen Elizabeth asked a seemingly simple but pointed question to a room of researchers and economists at the London School of Economics: Why did no one see it coming?
In the face of great complexity, perhaps econometrics could do with more help. Take machine learning for example. With its ability to parse big data, it could improve on existing econometric methods and lead to better forecasts. This is the research that Professor Yu Jun of the Singapore Management University (SMU) presented along with Associate Professor Xie Tian of the Shanghai University of Finance and Economics, at a webinar organized by SMU and the Monetary Authority of Singapore (MAS) on 26 June 2020.
In their talk titled "Econometric Methods and Data Science Techniques," Professors Yu and Xie reviewed existing econometric methods and machine learning techniques before discussing a hybrid of both methods. Using real data and examples, they showed that the hybrid method may herald better economic and financial variable forecasting.
"We all know that we are in the era of big data and machine learning data science techniques," said Professor Yu. "Some people may think that machine learning poses a threat to conventional econometric methods. Is that really the case?"
A mix of tradition and change
Speaking first, Professor Yu introduced a selection of traditional econometric methods. Blending economics with statistics, econometrics takes a structured quantitative statistical approach to economic analyses. With econometrics, Professor Yu explained, the method is to use past data to establish statistical relationships which in turn can be used to forecast possible futures.
"Most econometric methods hope to facilitate interpretation and statistical inference," he said, explaining that conventional econometric methods rely on assumptions and linear relationships, like the famous linearity assumption. "You want to map out from the past to the future."
This approach works well in certain cases, Professor Yu said, but also proves to be a limitation: most conventional econometric models cannot handle big data or complicated relationships. "If you have many predictors or a complicated relationship, econometric methods will fail. And that's a serious limitation in the big data era and in many important cases," he said.
On the other hand, Professor Xie, who is also an Adjunct Professor at SMU, explained that machine learning algorithms are data-driven. "Instead of relying on assumptions, many machine learning algorithms just let the data talk: they don't impose very strong assumptions or restrictions on the data-generating process," he said.
That's what makes machine learning techniques so flexible, Professor Xie pointed out. However, he also added that many machine learning methods are not truly tailored for economic and financial data in the first place.
So when it comes to trying to predict the future, is one approach better than the other? Like many things in this world, it's not so straightforward.
Weighing their strengths and weaknesses
Professors Yu and Xie used two real-world examples to illustrate how the two different approaches can outperform each other in accuracy depending on the data and case at hand.
In the forecasting of the Volatility Index, or VIX—an index of financial market volatility created by the Chicago Board Options Exchange—they showed that more traditional linear econometric modeling produced a more accurate forecast than more complex machine learning methods.
However, in the second case of forecasting the consumer price inflation of the eurozone, machine learning methods outperformed traditional econometric methods.
"Machine learning methods are very popular, but they do not always outperform conventional econometric methods. The question is, can we modify machine learning algorithms to adopt advanced econometric techniques and use economic data better?"
In this vein, Professors Yu and Xie discuss the idea that applying machine learning methods to existing econometrics approaches, instead of using either approach separately, could improve econometrics modeling. They suggest a hybrid algorithm, a model averaging regression tree (MART), which was first proposed by Professor Xie and Professor Steven F. Lehrer of Queen's University in a 2018 NBER Working Paper.
Putting MART to the test
To test the effectiveness of this hybrid method, they used it to forecast real economic and financial variables by applying it to the same examples of VIX and eurozone inflation rates discussed earlier.
Econometric models still had the best forecasting accuracy in forecasting VIX, performing better than their hybrid MART approach. Professors Yu and Xie suggest that this is because the VIX data exhibits very strong linearity and therefore is best suited for an econometric approach.
But when it came to forecasting the eurozone inflation rates, the results showed that the duo's hybrid approach performed best, generating superior forecast accuracy compared to either econometrics or machine learning methods alone.
So while a hybrid model isn't always the best in all cases, elements of machine learning may still improve on forecasting by picking up on trends that the traditional econometric models might miss. As for now, they say the key is to understand the fundamentals of each method and apply them in the most appropriate circumstances.
"The hybrid strategy combines econometric measures with machine learning strategies to lead to significant gains in forecasting accuracy," said Professor Xie. "Of course, this is just an idea. Future work is definitely needed to understand the properties of this proposed hybrid strategy in order to help guide practitioners."
Explore further Computers excel in chemistry class
Danish physicist Neils Bohr once quipped that prediction is hard, especially when it is about the future. But this is precisely what financial regulators need to do—forecasting the likely state of the economy in the future is crucial when deciding on policy levers like whether to slash or raise interest rates.
However, as the world continues to become more unpredictable, forecasting has become increasingly difficult. This challenge was poignantly illustrated after the start of the 2008 Financial Crisis, when Queen Elizabeth asked a seemingly simple but pointed question to a room of researchers and economists at the London School of Economics: Why did no one see it coming?
In the face of great complexity, perhaps econometrics could do with more help. Take machine learning for example. With its ability to parse big data, it could improve on existing econometric methods and lead to better forecasts. This is the research that Professor Yu Jun of the Singapore Management University (SMU) presented along with Associate Professor Xie Tian of the Shanghai University of Finance and Economics, at a webinar organized by SMU and the Monetary Authority of Singapore (MAS) on 26 June 2020.
In their talk titled "Econometric Methods and Data Science Techniques," Professors Yu and Xie reviewed existing econometric methods and machine learning techniques before discussing a hybrid of both methods. Using real data and examples, they showed that the hybrid method may herald better economic and financial variable forecasting.
"We all know that we are in the era of big data and machine learning data science techniques," said Professor Yu. "Some people may think that machine learning poses a threat to conventional econometric methods. Is that really the case?"
A mix of tradition and change
Speaking first, Professor Yu introduced a selection of traditional econometric methods. Blending economics with statistics, econometrics takes a structured quantitative statistical approach to economic analyses. With econometrics, Professor Yu explained, the method is to use past data to establish statistical relationships which in turn can be used to forecast possible futures.
"Most econometric methods hope to facilitate interpretation and statistical inference," he said, explaining that conventional econometric methods rely on assumptions and linear relationships, like the famous linearity assumption. "You want to map out from the past to the future."
This approach works well in certain cases, Professor Yu said, but also proves to be a limitation: most conventional econometric models cannot handle big data or complicated relationships. "If you have many predictors or a complicated relationship, econometric methods will fail. And that's a serious limitation in the big data era and in many important cases," he said.
On the other hand, Professor Xie, who is also an Adjunct Professor at SMU, explained that machine learning algorithms are data-driven. "Instead of relying on assumptions, many machine learning algorithms just let the data talk: they don't impose very strong assumptions or restrictions on the data-generating process," he said.
That's what makes machine learning techniques so flexible, Professor Xie pointed out. However, he also added that many machine learning methods are not truly tailored for economic and financial data in the first place.
So when it comes to trying to predict the future, is one approach better than the other? Like many things in this world, it's not so straightforward.
Weighing their strengths and weaknesses
Professors Yu and Xie used two real-world examples to illustrate how the two different approaches can outperform each other in accuracy depending on the data and case at hand.
In the forecasting of the Volatility Index, or VIX—an index of financial market volatility created by the Chicago Board Options Exchange—they showed that more traditional linear econometric modeling produced a more accurate forecast than more complex machine learning methods.
However, in the second case of forecasting the consumer price inflation of the eurozone, machine learning methods outperformed traditional econometric methods.
"Machine learning methods are very popular, but they do not always outperform conventional econometric methods. The question is, can we modify machine learning algorithms to adopt advanced econometric techniques and use economic data better?"
In this vein, Professors Yu and Xie discuss the idea that applying machine learning methods to existing econometrics approaches, instead of using either approach separately, could improve econometrics modeling. They suggest a hybrid algorithm, a model averaging regression tree (MART), which was first proposed by Professor Xie and Professor Steven F. Lehrer of Queen's University in a 2018 NBER Working Paper.
Putting MART to the test
To test the effectiveness of this hybrid method, they used it to forecast real economic and financial variables by applying it to the same examples of VIX and eurozone inflation rates discussed earlier.
Econometric models still had the best forecasting accuracy in forecasting VIX, performing better than their hybrid MART approach. Professors Yu and Xie suggest that this is because the VIX data exhibits very strong linearity and therefore is best suited for an econometric approach.
But when it came to forecasting the eurozone inflation rates, the results showed that the duo's hybrid approach performed best, generating superior forecast accuracy compared to either econometrics or machine learning methods alone.
So while a hybrid model isn't always the best in all cases, elements of machine learning may still improve on forecasting by picking up on trends that the traditional econometric models might miss. As for now, they say the key is to understand the fundamentals of each method and apply them in the most appropriate circumstances.
"The hybrid strategy combines econometric measures with machine learning strategies to lead to significant gains in forecasting accuracy," said Professor Xie. "Of course, this is just an idea. Future work is definitely needed to understand the properties of this proposed hybrid strategy in order to help guide practitioners."
Explore further Computers excel in chemistry class
Provided by Singapore Management University
No comments:
Post a Comment