DeepMind's MuZero conquers and learns the rules as it does
by Peter Grad , Tech Xplore
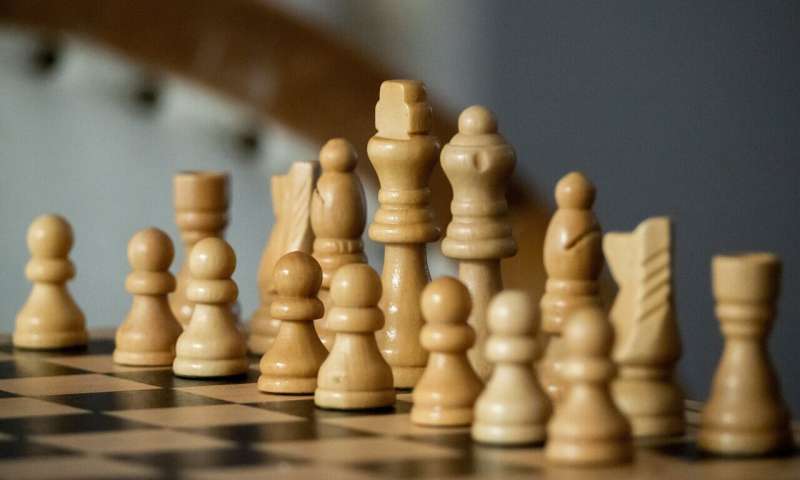
Credit: Unsplash/CC0 Public Domain
Albert Einstein once said, "You have to learn the rules of the game, and then you have to play better than anyone else." That could well be the motto at DeepMind, as a new report reveals it has developed a program that can master complex games without even knowing the rules.
DeepMind, a subsidiary of Alphabet, has previously made groundbreaking strides using reinforcement learning to teach programs to master the Chinese board game Go and the Japanese strategy game Shogi, as well as chess and challenging Atari video games. In all those instances, computers were given the rules of the game.
But Nature reported today that DeepMind's MuZero has accomplished the same feats—and in some instances, beat the earlier programs—without first learning the rules.
Programmers at DeepMind relied on a principle called "look-ahead search." With that approach, MuZero assesses a number of potential moves based on how an opponent would respond. While there would likely be a staggering number of potential moves in complex games such as chess, MuZero prioritizes the most relevant and most likely maneuvers, learning from successful gambits and avoiding ones that failed.
When performing against Atari's Ms. Pac-Man, MuZero was restricted to considering only six or seven potential future moves, yet still performed admirably, according to researchers.
"For the first time, we actually have a system that is able to build its own understanding of how the world works and use that understanding to do this kind of sophisticated look-ahead planning that you've previously seen for games like chess," said DeepMind's principal research scientist David Silver. MuZero can "start from nothing, and just through trial and error, both discover the rules of the world and use those rules to achieve kind of superhuman performance."
Silver envisions greater applications for MuZero than mere games. Progress has already been made on video compression, a challenging task considering the huge number of varying video formats and numerous modes of compression. So far, they have achieved a 5% improvement in compression, no small feat for the company owned by Google, which also handles the gigantic cache of videos on the world's second-most popular web site, YouTube, where a billion hours of content are viewed daily. (The No. 1 web site? Google.)
Silver says the laboratory is also looking into robotics programming and protein architecture design, which holds promise for personalized production of drugs.
It is a "significant step forward," according to Wendy Hall, professor of computer science at the University of Southampton and a member of England's AI council. "The results of DeepMind's work are quite astounding and I marvel at what they are going to be able to achieve in the future given the resources they have available to them," she said.
But she also raised a concern about the potential of abuse. "My worry is that whilst constantly striving to improve the performance of their algorithms and apply the results for the benefit of society, the teams at DeepMind are not putting as much effort into thinking through potential unintended consequences of their work," she said.
In fact, the U.S. Air Force had tapped early research papers covering MuZero that were made public last year and used the information to design an AI system that could launch missiles from a U-2 spy plane against specified targets.
When asked by Wired what he thought of such military applications, Silver left no doubt about his concerns.
"I oppose the use of AI in any deadly weapon, and I wish we had made more progress toward a ban on lethal autonomous weapons," he said. He added that DeepMind and its co-founders have all signed the Lethal Autonomous Weapons Pledge, which asserts the belief that deadly technology should always remain under human control, and not AI-based algorithms.
Silver says the challenges ahead are to understand and implement algorithms as effective and powerful as the human brain. "We should be aiming to achieve that. The first step in taking that journey is to try to understand what it even means to achieve intelligence," he said. "We think this really matters for enriching what AI can actually do because the world is a messy place. It's unknown—no one gives us this amazing rulebook that says, "Oh, this is exactly how the world works,'" Silver said. "If we want our AI to go out there into the world and be able to plan and look ahead in problems where no one gives us the rulebook, we really, really need this."
Explore further Alphabet's DeepMind masters Atari games
Albert Einstein once said, "You have to learn the rules of the game, and then you have to play better than anyone else." That could well be the motto at DeepMind, as a new report reveals it has developed a program that can master complex games without even knowing the rules.
DeepMind, a subsidiary of Alphabet, has previously made groundbreaking strides using reinforcement learning to teach programs to master the Chinese board game Go and the Japanese strategy game Shogi, as well as chess and challenging Atari video games. In all those instances, computers were given the rules of the game.
But Nature reported today that DeepMind's MuZero has accomplished the same feats—and in some instances, beat the earlier programs—without first learning the rules.
Programmers at DeepMind relied on a principle called "look-ahead search." With that approach, MuZero assesses a number of potential moves based on how an opponent would respond. While there would likely be a staggering number of potential moves in complex games such as chess, MuZero prioritizes the most relevant and most likely maneuvers, learning from successful gambits and avoiding ones that failed.
When performing against Atari's Ms. Pac-Man, MuZero was restricted to considering only six or seven potential future moves, yet still performed admirably, according to researchers.
"For the first time, we actually have a system that is able to build its own understanding of how the world works and use that understanding to do this kind of sophisticated look-ahead planning that you've previously seen for games like chess," said DeepMind's principal research scientist David Silver. MuZero can "start from nothing, and just through trial and error, both discover the rules of the world and use those rules to achieve kind of superhuman performance."
Silver envisions greater applications for MuZero than mere games. Progress has already been made on video compression, a challenging task considering the huge number of varying video formats and numerous modes of compression. So far, they have achieved a 5% improvement in compression, no small feat for the company owned by Google, which also handles the gigantic cache of videos on the world's second-most popular web site, YouTube, where a billion hours of content are viewed daily. (The No. 1 web site? Google.)
Silver says the laboratory is also looking into robotics programming and protein architecture design, which holds promise for personalized production of drugs.
It is a "significant step forward," according to Wendy Hall, professor of computer science at the University of Southampton and a member of England's AI council. "The results of DeepMind's work are quite astounding and I marvel at what they are going to be able to achieve in the future given the resources they have available to them," she said.
But she also raised a concern about the potential of abuse. "My worry is that whilst constantly striving to improve the performance of their algorithms and apply the results for the benefit of society, the teams at DeepMind are not putting as much effort into thinking through potential unintended consequences of their work," she said.
In fact, the U.S. Air Force had tapped early research papers covering MuZero that were made public last year and used the information to design an AI system that could launch missiles from a U-2 spy plane against specified targets.
When asked by Wired what he thought of such military applications, Silver left no doubt about his concerns.
"I oppose the use of AI in any deadly weapon, and I wish we had made more progress toward a ban on lethal autonomous weapons," he said. He added that DeepMind and its co-founders have all signed the Lethal Autonomous Weapons Pledge, which asserts the belief that deadly technology should always remain under human control, and not AI-based algorithms.
Silver says the challenges ahead are to understand and implement algorithms as effective and powerful as the human brain. "We should be aiming to achieve that. The first step in taking that journey is to try to understand what it even means to achieve intelligence," he said. "We think this really matters for enriching what AI can actually do because the world is a messy place. It's unknown—no one gives us this amazing rulebook that says, "Oh, this is exactly how the world works,'" Silver said. "If we want our AI to go out there into the world and be able to plan and look ahead in problems where no one gives us the rulebook, we really, really need this."
Explore further Alphabet's DeepMind masters Atari games
More information: Julian Schrittwieser et al. Mastering Atari, Go, chess and shogi by planning with a learned model, Nature (2020). DOI: 10.1038/s41586-020-03051-4
Journal information: Nature
No comments:
Post a Comment