Examining the link between body image and income
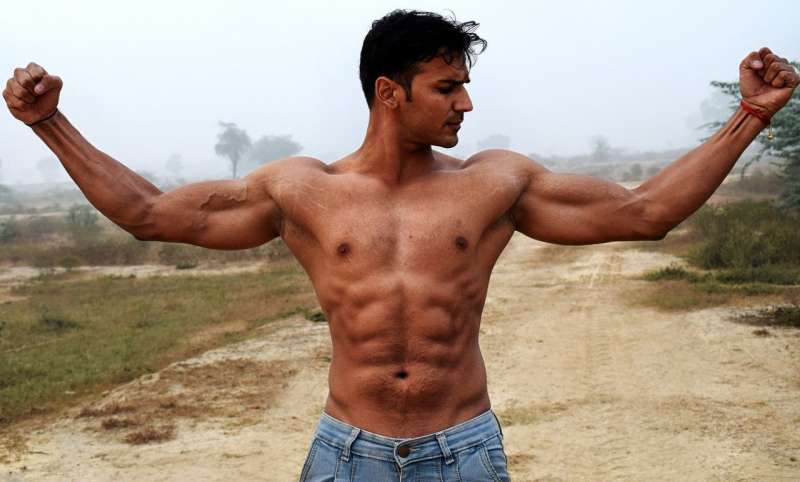
What started as a casual dinner conversation between two very different researchers in 2016—one a data scientist and engineer, the other an expert in economic models—has since turned into a journal article quantifying the effects of the "beauty premium," the notion that those who are more physically attractive tend to have a greater income.
The research team's engineer is Stephen Baek, an associate professor of data science at the University of Virginia, while the econometrician is Suyong Song, an associate professor of economics and finance at the University of Iowa. Five years ago, the two found that their research interests overlapped more than they initially realized, causing an unexpected idea to spark.
Baek began his collaboration with Song as a researcher at Iowa before joining the UVA School of Data Science faculty in August 2021. In his previous work, Baek analyzed and modeled human body shapes for engineering applications such as product design, virtual fashion, garment design and ergonomics. Song, on the other hand, brought expertise studying economic models that suffer from measurement and reporting error.
Compared to previous publications on the beauty premium, Baek and Song's research methods are novel, due to the nature of their data set, sourced from the 2002 Civilian American and European Surface Anthropometry Resource project, or CAESAR. In addition to self-reported height and weight measures—which have been used in previous studies—the project also gathered 3D body-scanned data, extensive information on demographic and family income, as well as tape-measure and caliper body measurements from nearly 2,400 civilians. With this data, the two researchers could provide a richer story of physical appearance and socio-economic variables.
"The issue with previous works was that people were oversimplifying the parameters to describe body shape," Baek said. "The traditional processes for determining physical appearance, such as stature, weight and BMI, are imperfect processes, and therefore not capable of capturing all the dimensions of human body shape."
Using a novel machine-learning algorithm called a "graphical autoencoder" or "deep machine learning," the 3D scans were inputted to encode geometric features of human body shape. After the machine was introduced to thousands of individual scans, the algorithm reduced the data's dimensionality—from a few hundreds of thousands of points down to a few important features –characterizing each human body shape using numerical values. Baek and Song then visualized the features to determine which body parts the algorithm was referencing and estimated their relations with socio-economic variables. Using this scientific approach, the causal effects of physical appearance could be quantified
For male and female subsamples, stature and obesity were both important features, while hip-to-waist ratio was an additional unique feature in the physical appearance of women. The empirical results found that greater stature in males was correlated to higher family income, while greater obesity in women was correlated to lower family income.
In addition to their findings regarding the beauty premium, Song's expertise in economic models added another layer to their findings: the negative role that survey and measurement error play in studies utilizing body measurements. According to his calculations—made possible by the fact that the 2002 data also included self-reported body measurements—Song found that reporting error highly correlated with true weight and height. On average, lighter-weight individuals tended to over-report their weight, whereas heavier individuals tended to under-report. The findings proved that survey errors regarding these measurements are substantial, and that previous studies utilizing self-reported survey data likely suffer because of it. Song explained that when regression models are run in which economic variables suffer from survey or measurement error, the estimation becomes biased, blurring the correct relationship.
"To address the issue of error, many economists assume that these errors are negligible or they are zero on average," Song said. "However, our study showed that they are not negligible and they are not zero on average, but rather showed that they are correlated with true height or weight, which alarms many studies using survey data."
Initially, Song anticipated a target audience of economists and statisticians, but with these findings, has since realized the topic's broader impact on fields like engineering, computer science, biology and social science.
Three years after its initial submission, the research paper, "Body Shape Matters: Evidence from Machine Learning on Body Shape-Income Relationship," was published in the open-access journal, PLOS One.
With heightened publicity, not only do Baek and Song hope to present the extent of error in previous body shape studies that relied on self-reported survey data, but also to bring awareness to the issue of beauty premiums.Breakthrough in 3D scanning leads to 4500% more accurate results
More information: Suyong Song et al, Body shape matters: Evidence from machine learning on body shape-income relationship, PLOS ONE (2021). DOI: 10.1371/journal.pone.0254785
Journal information: PLoS ONE
Provided by University of Virginia
No comments:
Post a Comment