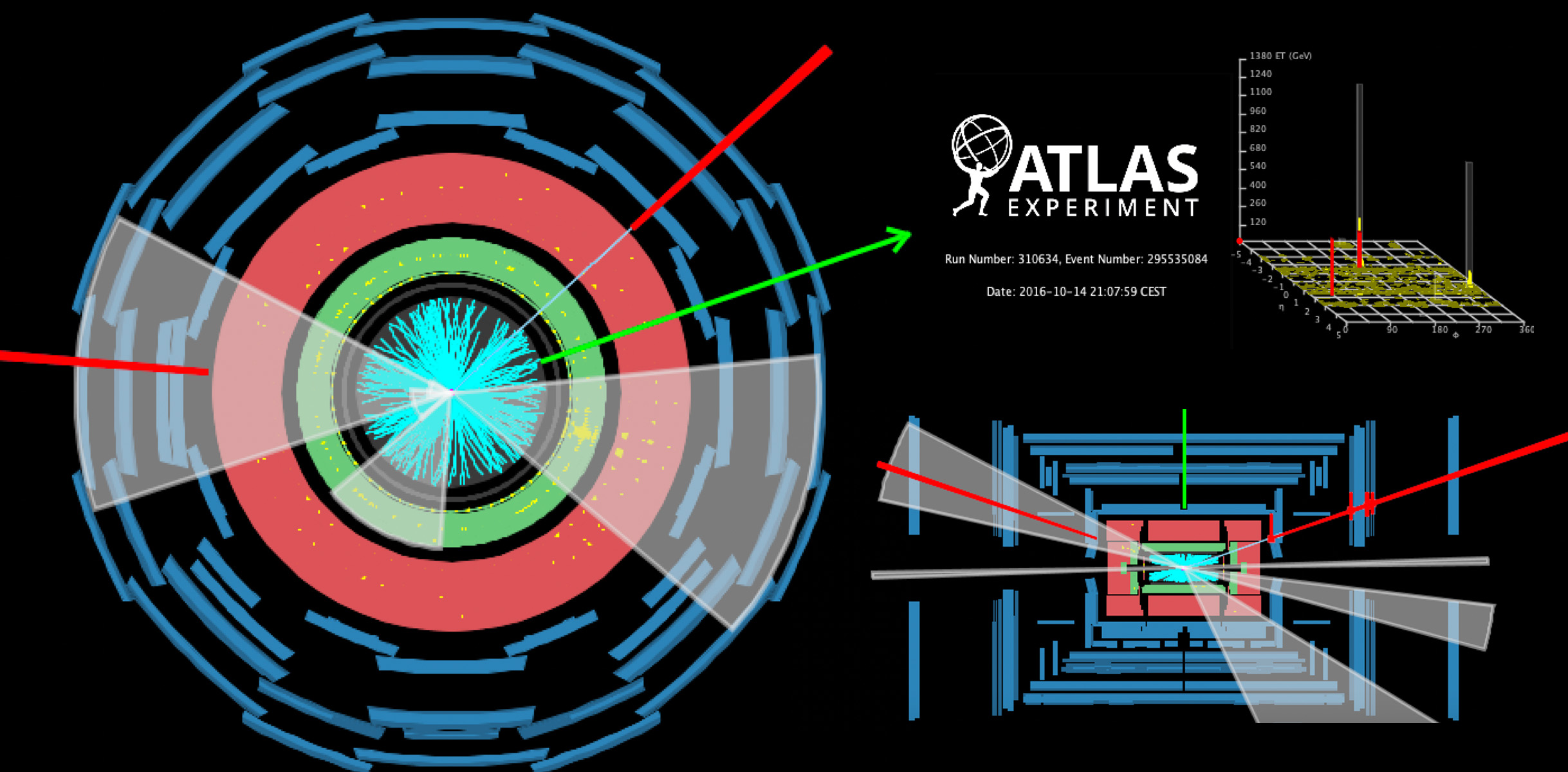
24 August 2023 |
ATLAS Collaboration
Since starting up in 2009, the Large Hadron Collider (LHC) has been at the forefront of scientific exploration – with researchers driven to uncover new particles and phenomena that go beyond the Standard Model. Over the years, thousands of scientists have channelled their expertise into refining analysis techniques and developing new ways to find these new-physics phenomena.
Since starting up in 2009, the Large Hadron Collider (LHC) has been at the forefront of scientific exploration – with researchers driven to uncover new particles and phenomena that go beyond the Standard Model. Over the years, thousands of scientists have channelled their expertise into refining analysis techniques and developing new ways to find these new-physics phenomena.
Figure 1: A schematic representation of the autoencoder architecture used for training and selection of the three anomaly regions. (Image: ATLAS Collaboration)
Traditionally, searches for new physics use complex computer simulations to reproduce what Standard Model processes should look like in collisions recorded by the ATLAS Experiment. These are then compared to simulations of new-physics models (e.g. dark matter, supersymmetry, etc.). Such models also help physicists determine the types of collisions where new-physics processes would be very prominent or where the collisions cannot be described by Standard-Model simulations – thus focusing their searches for new phenomena. Another style of searches involves looking at small deviations to a Standard-Model background caused by possible new phenomena.
Unsupervised machine learning can offer a new style of analyses which is completely agnostic to types of new-physics models and to any expectations of scientists. Researchers can design a complex neural network with millions of interconnections between “neurons”, and train this network on real data (see Figure 1). After training, the neural network can recognise “typical” LHC collisions and filter them out, leaving behind only the unrecognised or “atypical” collision events. On a technical side, such an unsupervised deep neural network (called an autoencoder) compresses input information, and then decompresses it while comparing inputs with outputs. Events with large reconstruction differences are called an “anomaly” since the algorithm finds itself in “trouble” in identifying such events. The chances that the anomalous events belong to new-physics phenomena are high. When using such neural networks, the idea is to look at the anomalous events, reconstruct the invariant masses of the particles in the collision, and then decide if they can be described by a Standard-Model process.
The new ATLAS result pioneers the use of unsupervised machine learning to search for anomalous collision events which could be from new-physics phenomena.
Traditionally, searches for new physics use complex computer simulations to reproduce what Standard Model processes should look like in collisions recorded by the ATLAS Experiment. These are then compared to simulations of new-physics models (e.g. dark matter, supersymmetry, etc.). Such models also help physicists determine the types of collisions where new-physics processes would be very prominent or where the collisions cannot be described by Standard-Model simulations – thus focusing their searches for new phenomena. Another style of searches involves looking at small deviations to a Standard-Model background caused by possible new phenomena.
Unsupervised machine learning can offer a new style of analyses which is completely agnostic to types of new-physics models and to any expectations of scientists. Researchers can design a complex neural network with millions of interconnections between “neurons”, and train this network on real data (see Figure 1). After training, the neural network can recognise “typical” LHC collisions and filter them out, leaving behind only the unrecognised or “atypical” collision events. On a technical side, such an unsupervised deep neural network (called an autoencoder) compresses input information, and then decompresses it while comparing inputs with outputs. Events with large reconstruction differences are called an “anomaly” since the algorithm finds itself in “trouble” in identifying such events. The chances that the anomalous events belong to new-physics phenomena are high. When using such neural networks, the idea is to look at the anomalous events, reconstruct the invariant masses of the particles in the collision, and then decide if they can be described by a Standard-Model process.
The new ATLAS result pioneers the use of unsupervised machine learning to search for anomalous collision events which could be from new-physics phenomena.
Figure 2: Example of the invariant mass (jet+muon) in the anomalous region defined by the unsupervised machine learning algorithm trained on a fraction of real data. The fit is represented by the red line, while the associated statistical uncertainties are indicated by the shaded band.The lower panel shows the bin-by-bin significances of deviations from the fit. (Image: ATLAS Collaboration)
In a new paper submitted to Phys. Rev. Lett., the ATLAS Collaboration pioneers the use of this style of physics analysis using LHC Run-2 data (collected 2015-2018). This analysis is the first of its kind, and marks the inaugural application of this type of unsupervised machine learning at a collider experiment, whether at the LHC or elsewhere.
ATLAS physicists observed no significant deviations from the Standard Model in the anomaly regions. The largest deviation was found for a mass at around 4.8 TeV with a significance of about 2.9 sigma for one decay channel (Figure 2). This level of statistical confidence typically means that the experimental observation could be a promising hint, but not sufficient for claiming the observation. The event display in the header of this briefing illustrates a typical collision event in the anomaly region with the jet+muon mass where the largest deviation is observed.
This analysis technique offers a new paradigm for searching for new-physics phenomena. One that relies less on wondering how the “new” phenomena may look, and instead focusing on new and unexpected model-agnostic signatures. In short, continuing the decade-long tradition of LHC physicists to discover a path into the unexplored realms of physics.About the event display: A display of an event with the reconstructed invariant mass of 4.72 TeV in the anomaly region as reported by the autoencoder trained using ATLAS data. The grey cones represent jets and the red lines represent muons. The green arrow indicates a missing transverse energy (MET). The red line closest to MET represents a high-energy muon. (Image: ATLAS Collaboration)
In a new paper submitted to Phys. Rev. Lett., the ATLAS Collaboration pioneers the use of this style of physics analysis using LHC Run-2 data (collected 2015-2018). This analysis is the first of its kind, and marks the inaugural application of this type of unsupervised machine learning at a collider experiment, whether at the LHC or elsewhere.
ATLAS physicists observed no significant deviations from the Standard Model in the anomaly regions. The largest deviation was found for a mass at around 4.8 TeV with a significance of about 2.9 sigma for one decay channel (Figure 2). This level of statistical confidence typically means that the experimental observation could be a promising hint, but not sufficient for claiming the observation. The event display in the header of this briefing illustrates a typical collision event in the anomaly region with the jet+muon mass where the largest deviation is observed.
This analysis technique offers a new paradigm for searching for new-physics phenomena. One that relies less on wondering how the “new” phenomena may look, and instead focusing on new and unexpected model-agnostic signatures. In short, continuing the decade-long tradition of LHC physicists to discover a path into the unexplored realms of physics.About the event display: A display of an event with the reconstructed invariant mass of 4.72 TeV in the anomaly region as reported by the autoencoder trained using ATLAS data. The grey cones represent jets and the red lines represent muons. The green arrow indicates a missing transverse energy (MET). The red line closest to MET represents a high-energy muon. (Image: ATLAS Collaboration)
Learn more
Search for new phenomena in two-body invariant mass distributions using unsupervised machine learning for anomaly detection at 13 TeV with the ATLAS detector (arXiv:2307.01612, see figures)
EPS-HEP 2023 poster by Alkaid Cheng:
EPS-HEP 2023 poster by Alkaid Cheng:
Search for new physics using unsupervised machine learning for anomaly detection in 13 TeV collisions recorded by the ATLAS detector at the LHC
LHCP 2023 parallel talk by Rui Zhang:
LHCP 2023 parallel talk by Rui Zhang:
Search for new physics using unsupervised machine learning for anomaly detection with ATLAS
BOOST 2023 parallel talk by Wasikul Islam:
BOOST 2023 parallel talk by Wasikul Islam:
No comments:
Post a Comment