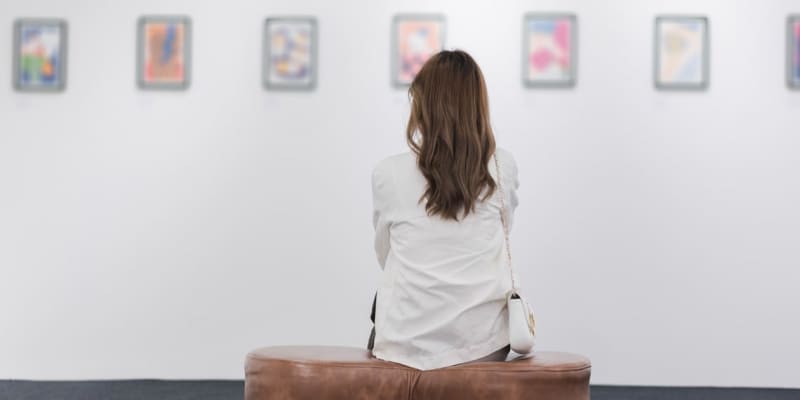
A team of scientists recently utilized generative AI software to demonstrate a strong link between self-relevance and what people find visually attractive in art. The findings, published in Psychological Science, provide evidence that when a piece of art is connected to something meaningful in our own lives, we’re more likely to perceive it as aesthetically appealing, independent of the specific qualities of the artwork itself.
Aesthetic judgments of faces and natural landscapes tend to be relatively consistent across individuals, but shared taste in art accounts for only a small percentage (10% to 20%) of reliable variance in aesthetic ratings. This suggests that there is a substantial amount of variability in how people perceive and appreciate art.
The researchers hypothesized that an essential factor in determining the aesthetic appeal of art is its capacity to resonate with an individual’s self-construct. They propose that artworks that speak to a person’s self-schema, which includes their self-perception, past experiences, and personal identity, are more likely to be aesthetically appealing to that individual.
“Aesthetics affect so many aspects of our lives. Not only what we buy, who we spend time with, or where we live or go on vacation, but also in small and big ways every day,” said study author Edward A. Vessel, the Eugene Surowitz Assistant Professor of Psychology at the City College of New York.
“The aesthetics of our environment affect how we feel, how we heal, and how we relate. Aesthetics reflect more than just whether something is “pretty”, but really tell us about how well the world fits, or doesn’t fit, our _model_ of the world.”
“There’s been a flurry of work recently showing that when you use the most sophisticated machine-learning models available, you can predict a degree of aesthetic appeal from the features of images (though I would note that even in this work, those ‘features’ that are most predictable are in fact really-high level meanings that we, as a culture, agree on). But it’s clear that, especially with artwork, people can have really unique tastes that differ a lot from ‘average’ ratings of appeal.”
“We wanted to try to get inside people’s heads and understand what internal representations lead to such divergent aesthetic tastes. This led us to start doing research on self-relevance: how much a particular image or experience relates to your self-construct.”
The researchers conducted a series of three experiments to investigate the relationship between self-relevance and aesthetic appeal.
The first two experiments, which included 33 German-speaking participants recruited through a research participant database maintained by the Max Planck Institute for Empirical Aesthetics and 208 English-speaking participants recruited online via Prolific, aimed to establish whether there was a relationship between aesthetic ratings of visual art and self-relevance judgments.
In the first experiment, the participants viewed a diverse selection of artwork, spanning various time periods, styles, and cultures. After viewing each image, the participants indicated the extent to which something in the art related “to you, your experiences, or your identity” (self-relevance) and how “moved” they were by artwork (aesthetic appeal). The second experiment followed a similar procedure, with slightly different questions.
In both experiments, the researchers found a strong and positive relationship between individual ratings of self-relevance and aesthetic appeal. Despite differences in which artworks participants personally found appealing or self-relevant, the correlation between these two measures was robust.
Next, Vessel and his colleagues aimed to further investigate the relationship between self-relevance and aesthetic appeal using novel AI-created artworks. To do this, they used a deep convolutional neural network to perform style transfer on existing artworks, creating new synthetic artworks with personalized content based on each participant’s responses to a questionnaire. These new artworks were designed to be highly controlled for both content and style, without explicitly asking participants about self-relevance.
The experiment involved 40 German-speaking participants aged 18 to 55. The participants first completed a Cultural Background and Lifestyle Questionnaire that assessed “significant locations associated with autobiographical experiences, aspects of their personal identity, and personal interests in topics such as the arts, style, and cuisine.”
The participants were then paired with others whose questionnaire responses were sufficiently divergent to create self-relevant and other-relevant images. They then viewed and rated a total of 80 artworks, divided into four conditions:
Real Artwork: 20 paintings selected from a diverse set of styles, genres, periods, and cultural origins.
AI-Generated Control Artwork: 20 novel artworks created using the style-transfer algorithm. These were the same for all participants.
AI-Generated Self-Relevant Artwork: 20 artworks uniquely generated for each participant based on their responses to the Cultural Background and Lifestyle Questionnaire.
AI-Generated Other-Relevant Artwork: 20 artworks generated for each participant’s paired partner, with matching artistic styles but different content.
The researchers found that participants rated the AI-generated self-relevant artworks as significantly more self-relevant compared to other categories of artworks. This confirmed that the manipulation of self-relevance was successful.
Importantly, artwork generated from self-relevant content were consistently rated as significantly more aesthetically appealing than matched other-relevant artwork and AI-generated control artwork. This finding further supported the idea that the subjective, personal connection individuals have with artworks plays a crucial role in shaping their aesthetic judgments.
“The primary finding is pretty straightforward,” Vessel told PsyPost. “When an image contains self-relevant content, such as things that relate to memories about yourself, to how you identify, or to your core life experiences (e.g. where you grew up), a person tends to like it more. But someone else with different life experiences, will tend to like the same image less.”
“We think this works because self-relevant content acts like a map or a key. It allows a person to unlock deeper levels of meaning, even when the art is actually about someone else’s experience. And this makes the experience more pleasurable, because we are learning about the world, about ourselves, and about our relationship to the world.”
The researchers also classified AI-generated self-relevant artworks into different subclasses based on the nature of the questionnaire items from which they were derived. They found that artworks related to specific autobiographical memories, aspects of personal identity, expressed preferences, and interests were rated as most appealing. However, artworks related to common activities did not show the same effect.
“I think these results are really interesting for several reasons,” Vessel said. “In addition to their usefulness for understanding a basic aspect of human experience, they also point the way to how we might create more impactful art therapies, which are increasingly being recognized as an effective and cost efficient tool for addressing physical and mental health challenges.”
“But they also present a cautionary tale about the increasing use of AI by tech and media companies to generate self-relevant content from models of their consumers,” the researcher added. “Personalized content is hard to turn away from, and in this way, has the potential to be addictive and maladaptive. We need to recognize this potential for harm and consider public policies for protecting consumers from a digital version of Harry Potter’s ‘Mirror of Erised,’ serving us an idealized view of ourselves and our world.”
The study, “Self-Relevance Predicts the Aesthetic Appeal of Real and Synthetic Artworks Generated via Neural Style Transfer“, was authored by Edward A. Vessel, Laura Pasqualette, Cem Uran, Sarah Koldehoff, Giacomo Bignardi, and Martin Vinck.
2023/09/08
© PsyPost
No comments:
Post a Comment