Light it up: Using firefly genes to understand cannabis biology
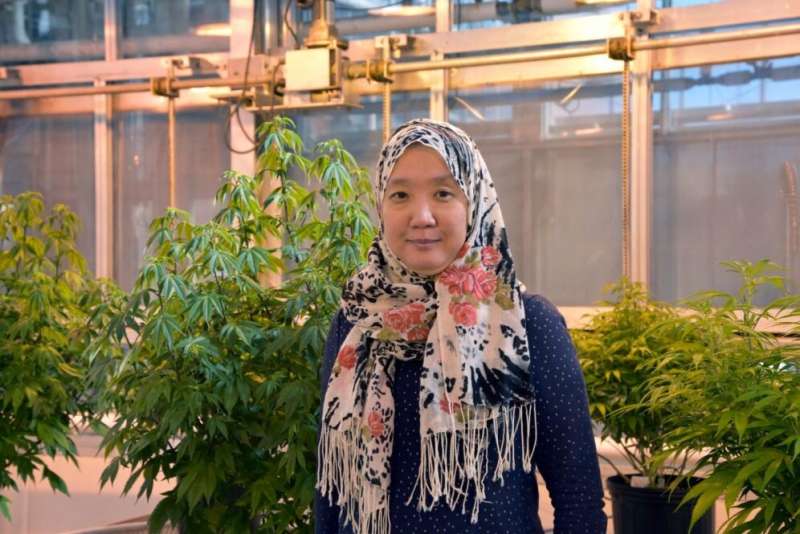
Cannabis, a plant gaining ever-increasing attention for its wide-ranging medicinal properties, contains dozens of compounds known as cannabinoids.
One of the best-known cannabinoids is cannabidiolic acid (CBD), which is used to treat pain, inflammation, nausea and more.
Cannabinoids are produced by trichomes, small spikey protrusions on the surface of cannabis flowers. Beyond this fact, scientists know very little about how cannabinoid biosynthesis is controlled.
Yi Ma, research assistant professor, and Gerry Berkowitz, professor in the College of Agriculture, Health and Natural Resources, investigated the underlying molecular mechanisms behind trichrome development and cannabinoid synthesis.
Berkowitz and Ma, along with former graduate students Samuel Haiden and Peter Apicella, discovered transcription factors responsible for trichome initiation and cannabinoid biosynthesis. Transcription factors are molecules that determine if a piece of an organism's DNA will be transcribed into RNA, and thus expressed.
In this case, the transcription factors cause epidermal cells on the flowers to morph into trichomes. The team's discovery was recently published as a feature article in Plants. Related trichome research was also published in Plant Direct. Due to the gene's potential economic impact, UConn has filed a provisional patent application on the technology.
Building on their results, the researchers will continue to explore how these transcription factors play a role in trichome development during flower maturation.
Berkowitz and Ma will clone the promoters (the part of DNA that transcription factors bind to) of interest. They will then put the promoters into the cells of a model plant along with a copy of the gene that makes fireflies light up, known as firefly luciferase; the luciferase is fused to the cannabis promoter so if the promoter is activated by a signal, the luciferase reporter will generate light. "It's a nifty way to evaluate signals that orchestrate cannabinoid synthesis and trichome development," says Berkowitz.
The researchers will load the cloned promoters and luciferase into a plasmid. Plasmids are circular DNA molecules that can replicate independently of the chromosomes. This allows the scientists to express the genes of interest even though they aren't part of the plant's genomic DNA. They will deliver these plasmids into the plant leaves or protoplasts, plant cells without the cell wall.
When the promoter controlling luciferase expression comes into contact with the transcription factors responsible for trichome development (or triggered by other signals such as plant hormones), the luciferase "reporter" will produce light. Ma and Berkowitz will use an instrument called a luminometer, which measures how much light comes from the sample. This will tell the researchers if the promoter regions they are looking at are controlled by transcription factors responsible for increasing trichome development or modulating genes that code for cannabinoid biosynthetic enzymes. They can also learn if the promoters respond to hormonal signals.
In prior work underlying the rationale for this experimental approach, Ma and Berkowitz along with graduate student Peter Apicella found that the enzyme that makes THC in cannabis trichomes may not be the critical limiting step regulating THC production, but rather the generation of the precursor for THC (and CBD) production and the transporter-facilitated shuttling of the precursor to the extracellular bulb might be key determinants in developing cannabis strains with high THC or CBD.
Most cannabis farmers grow hemp, a variety of cannabis with naturally lower THC levels than marijuana. Currently, most hemp varieties that have high CBD levels also contain unacceptably high levels of THC. This is likely because the hemp plants still make the enzyme that produces THC. If the plant contains over 0.3% THC, it is considered federally illegal and, in many cases, must be destroyed. A better understanding of how the plant produces THC means scientists could selectively knock out the enzyme that synthesizes THC using genome editing techniques such as CRISPR. This would produce plants with lower levels of or no THC.
"We envision that the fundamental knowledge obtained can be translated into novel genetic tools and strategies to improve the cannabinoid profile, aid hemp farmers with the common problem of overproducing THC, and benefit human health," the researchers say.
On the other hand, this knowledge could lead to the production of cannabis plants that produce more of a desired cannabinoid, making it more valuable and profitable.The frostier the flower, the more potent the cannabis
More information: Samuel R. Haiden et al, Overexpression of CsMIXTA, a Transcription Factor from Cannabis sativa, Increases Glandular Trichome Density in Tobacco Leaves, Plants (2022). DOI: 10.3390/plants11111519
Peter V. Apicella et al, Delineating genetic regulation of cannabinoid biosynthesis during female flower development in Cannabis sativa, Plant Direct (2022). DOI: 10.1002/pld3.412
Inexpensive method detects synthetic cannabinoids, banned pesticides

Scientists have modified proteins involved in plants' natural response to stress, making them the basis of innovative tests for multiple chemicals, including banned pesticides and deadly, synthetic cannabinoids.
During drought, plants produce ABA, a hormone that helps them hold on to water. Additional proteins, called receptors, help the plant recognize and respond to ABA. UC Riverside researchers helped demonstrate that these ABA receptors can be easily modified to quickly signal the presence of nearly 20 different chemicals.
The research team's work in transforming these plant-based molecules is described in a new Nature Biotechnology journal article.
Researchers frequently need to detect all kinds of molecules, including those that harm people or the environment. Though methods to do that exist, they are often costly and require complicated equipment.
"It would be transformative if we could develop rapid dipstick tests to know if a dangerous chemical, like a synthetic cannabinoid, is present. This new paper gives others a roadmap to doing that," said Sean Cutler, a UCR plant cell biology professor and paper co-author.
The problem with synthetic cannabinoids is something Cutler calls, "regulatory whack-a-mole." Because they send people to the hospital, authorities have attempted to outlaw them in this country. However, dozens of new versions emerge every year before they can be controlled.
"Our system could be configured to detect lab-made cannabinoid variations as quickly as they appear on the market," Cutler said.
The research team also demonstrated their testing system can signal the presence of organophosphates, which includes many banned pesticides that are toxic and potentially lethal to humans. Not all organophosphate pesticides are banned but being able to quickly detect the ones that are could help officials monitor water quality without more expensive testing at laboratories.
For this project, the researchers demonstrated the system in laboratory-grown yeast cells. In the future, the team would like to put the modified molecules back into plants that could serve as biological sensors. In that case, a chemical in the environment could cause leaves to turn specific colors or change temperatures.
Although the work focuses on cannabinoids and pesticides, the key breakthrough here is the ability to rapidly develop diagnostics for chemicals using a simple and inexpensive system. "If we can expand this to lots of other chemical classes, this is a big step forward because developing new tests can be a slow process," said Ian Wheeldon, study co-author and UCR chemical engineer.
This research was developed through a contract with the Donald Danforth Plant Science Center to support the Defense Advanced Research Projects Agency (DARPA) Advanced Plant Technologies (APT) program. The team included scientists from the Medical College of Wisconsin, Michigan State University, and the Donald Danforth Plant Science Center in St. Louis. This work was facilitated by chemical and biological engineer Timothy Whitehead at the University of Colorado, Boulder.
To create this system, researchers took advantage of the ABA plant stress hormone's ability to switch receptor molecules on and off. In the "on" position, the receptors bind to another protein, forming a tight complex that can trigger visible responses, like glowing. Whitehead, a collaborator on the work, used state-of-the-art computational tools to help redesign the receptors, which was critical to the success of the group's work.
"We take an enzyme that can glow in the right context and split it into two pieces. One piece on the switch, and the other on the protein it binds to," Cutler said. "This trick of bringing two things together in the presence of a third chemical isn't new. Our advance is showing we can reprogram the process to work with lots of different third chemicals."