Want to find UFOs? That's a job for machine learning
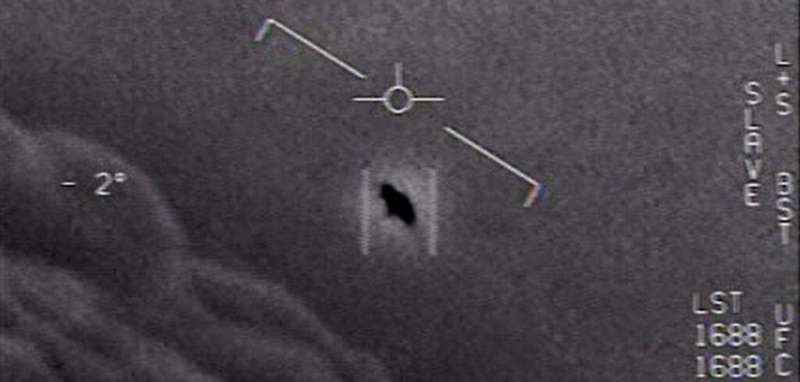
In 2017, humanity got its first glimpse of an interstellar object (ISO), known as 1I/"Oumuamua, which buzzed our planet on its way out of the solar system. Speculation abound as to what this object could be because, based on the limited data collected, it was clear that it was like nothing astronomers had ever seen. A controversial suggestion was that it might have been an extraterrestrial probe (or a piece of a derelict spacecraft) passing through our system.
Public fascination with the possibility of "alien visitors" was also bolstered in 2021 with the release of the UFO Report by the ODNI.
This move effectively made the study of unidentified aerial phenomena (UAP) a scientific pursuit rather than a clandestine affair overseen by government agencies. With one eye on the skies and the other on orbital objects, scientists are proposing how recent advances in computing, AI, and instrumentation can be used to assist in the detection of possible "visitors." This includes a recent study by a team from the University of Strathclyde that examines how hyperspectral imaging paired with machine learning can create an advanced data pipeline.
The team was led by Massimiliano Vasile, a professor of mechanical and aerospace engineering, and was composed of researchers from the schools of Mechanical and Aerospace Engineering and Electronic and Electrical Engineering at the University of Strathclyde and the Fraunhofer Center for Applied Photonics in Glasgow.
A preprint of their paper, titled "Space Object Identification and Classification from Hyperspectral Material Analysis," is available online via the pre-print server arXiv and is being reviewed for publication in Scientific Reports.
This study is the latest in a series that addresses applications for hyperspectral imaging for activities in space. The first paper, "Intelligent characterization of space objects with hyperspectral imaging," appeared in Acta Astronautica in February 2023 and was part of the Hyperspectral Imager for Space Surveillance and Tracking (HyperSST) project. This was one of 13 debris mitigation concepts selected by the UK Space Agency (UKSA) for funding last year and is the precursor to the ESA's Hyperspectral space debris Classification (HyperClass) project.
Their latest paper explored how this same imaging technique could be used in the growing field of UAP identification. This process consists of collecting and processing data from across the electromagnetic spectrum from single pixels, typically to identify different objects or materials captured in images. As Vasile explained to Universe Today via email, hyperspectral imaging paired with machine learning has the potential for narrowing the search for possible technosignatures by eliminating false positives caused by human-made debris objects (spent stages, defunct satellites, etc.):
"If UAP are space objects, then what we can do by analyzing the spectra is to understand the material composition even from a single pixel. We can also understand the attitude motion by analyzing the time variation of the spectra. Both things are very important because we can identify object by their spectral signature and understand their motion with minimal optical requirements."
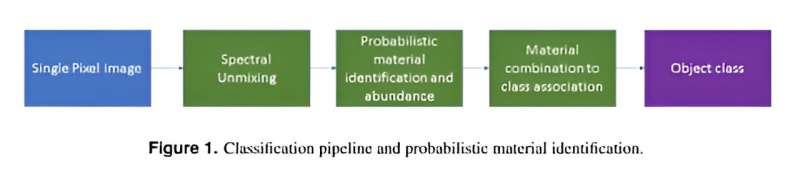
Vasile and his colleagues propose the creation of a data processing pipeline for processing UAP images using machine learning algorithms. As a first step, they explained how a dataset of time-series spectra of space objects is needed for the pipeline, including satellites and other objects in orbit. This includes debris objects, which means incorporating data from NASA's Orbital Debris Program Office (ODPO), the ESA's Space Debris Office, and other national and international bodies. This dataset must be diverse and include orbital scenarios, trajectories, illumination conditions, and precise data on the geometry, material distribution, and attitude motion of all orbiting objects at all times.
In short, scientists would need a robust database of all human-made objects in space for comparison to eliminate false positives. Since much of this data is unavailable, Vasile and his team created numerical physics simulation software to produce training data for the machine learning models. The next step involved a two-pronged approach to associate a spectrum to a set of materials generating it, one based on machine learning and one based on a more traditional mathematical regression analysis used to determine the line of best fit for a set of data (aka. least square method).
They then used a machine learning-based classification system to associate the probability of detecting a combination of materials with a particular class. With the pipeline complete, said Vasile, the next step was to run a series of tests, which provided encouraging data:
"We ran three tests: one in a laboratory with a mockup of a satellite made of known materials. These tests were very positive. Then we created a high-fidelity simulator to simulate real observation of objects in orbit. Test were positive and we learnt a lot. Finally we used a telescope and we observed a number of satellites and the space station. In this case, some tests were good some less good because our material database is currently rather small."
In their next paper, Vasile and his colleagues will present the attitude reconstruction part of their pipeline, which they hope to present at the upcoming AIAA Science and Technology Forum and Exposition (2024 SciTech) from January 8th to 12th in Orlando, Florida.
More information: Massimiliano Vasile et al, Space Object Identification and Classification from Hyperspectral Material Analysis, arXiv (2023). DOI: 10.48550/arxiv.2308.07481
Journal information: Scientific Reports , arXiv , Acta Astronautica
Provided by Universe Today Detecting and estimating satellite maneuvers more accurately