How the Brain Makes Memories and Uses “Time Travel” To Remember
Cedars-Sinai researchers discover neurons that separate experience into segments, then help the brain ‘time travel’ and remember.
In a study led by Cedars-Sinai, researchers have discovered two types of brain cells that play a key role in dividing continuous human experience into distinct segments that can be recalled later. The discovery provides new promise as a path toward development of novel treatments for memory disorders such as dementia and Alzheimer’s disease.
The study, part of a multi-institutional BRAIN Initiative consortium funded by the National Institutes of Health and led by Cedars-Sinai, was published in the peer-reviewed journal Nature Neuroscience. As part of ongoing research into how memory works, Ueli Rutishauser, PhD, professor of Neurosurgery, Neurology, and Biomedical Sciences at Cedars-Sinai, and co-investigators looked at how brain cells react as memories are formed.
“One of the reasons we can’t offer significant help for somebody who suffers from a memory disorder is that we don’t know enough about how the memory system works,” said Rutishauser, senior author of the study, adding that memory is foundational to us as human beings.
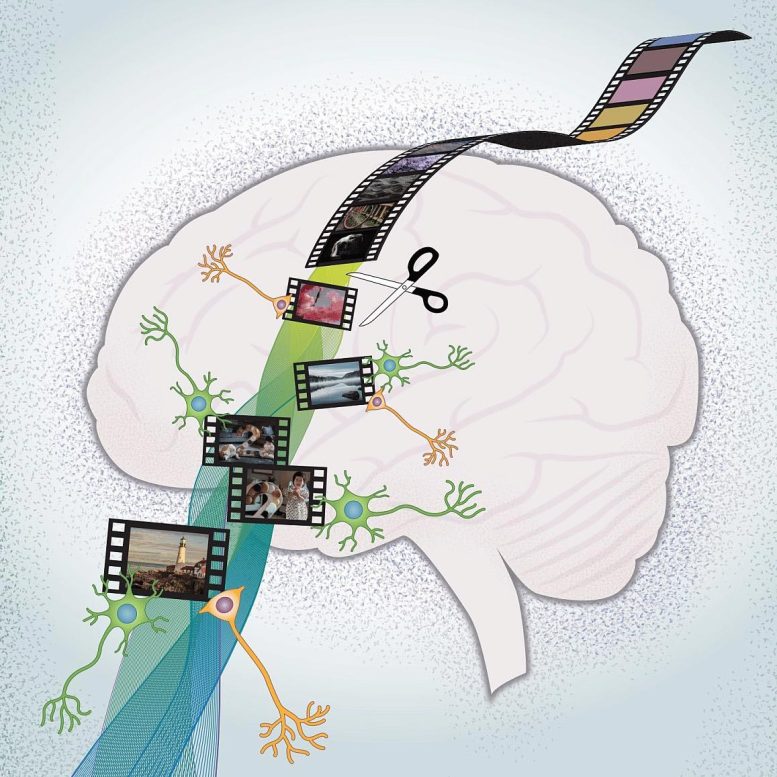
Researchers have discovered two types of brain cells that play a key role in creating memories. Credit: Sarah Pyle for Cedars-Sinai Medical Center
Human experience is continuous, but psychologists believe, based on observations of people’s behavior, that memories are divided by the brain into distinct events, a concept known as event segmentation. Working with 19 patients with drug-resistant epilepsy, Rutishauser and his team were able to study how neurons perform during this process.
Patients participating in the study had electrodes surgically inserted into their brains to help locate the focus of their epileptic seizures, allowing investigators to record the activity of individual neurons while the patients viewed film clips that included cognitive boundaries.
While these boundaries in daily life are nuanced, for research purposes, the investigators focused on “hard” and “soft” boundaries.
“An example of a soft boundary would be a scene with two people walking down a hallway and talking, and in the next scene, a third person joins them, but it is still part of the same overall narrative,” said Rutishauser, interim director of the Center for Neural Science and Medicine and the Board of Governors Chair in Neurosciences at Cedars-Sinai.
In the case of a hard boundary, the second scene might involve a completely different set of people riding in a car. “The difference between hard and soft boundaries is in the size of the deviation from the ongoing narrative,” Rutishauser said. “Is it a totally different story, or like a new scene from the same story?”
When study participants watched film clips, investigators noted that certain neurons in the brain, which they labeled “boundary cells,” increased their activity after both hard and soft boundaries. Another group of neurons, labeled “event cells,” increased their activity only in response to hard boundaries, but not soft boundaries.
Rutishauser and his co-investigators theorize that peaks in the activity of boundary and event cells—which are highest after hard boundaries, when both types of cells fire—send the brain into the proper state for initiating a new memory.
“A boundary response is kind of like creating a new folder on your computer,” said Rutishauser. “You can then deposit files in there. And when another boundary comes around, you close the first folder and create another one.”
To retrieve memories, the brain uses boundary peaks as what Rutishauser calls “anchors for mental time travel.”
“When you try to remember something, it causes brain cells to fire,” Rutishauser said. “The memory system then compares this pattern of activity to all the previous firing peaks that happened shortly after boundaries. If it finds one that is similar, it opens that folder. You go back for a few seconds to that point in time, and things that happened then come into focus.”
To test their theory, investigators gave study participants two memory tests.
They first showed participants a series of still images and asked them whether or not they had seen them in the film clips they had viewed. Study participants were more likely to remember images that closely followed a hard or soft boundary, when a new “memory folder” would have been created.
Investigators also showed participants pairs of images from film clips they had viewed and asked which of the images appeared first. Participants had difficulty remembering the correct order of images that appeared on opposite sides of a hard boundary, possibly because the brain had segmented those images into separate memory folders.
Rutishauser said that therapies that improve event segmentation could help patients with memory disorders. Even something as simple as a change in atmosphere can amplify event boundaries, he explained.
“The effect of context is actually quite strong,” Rutishauser said. “If you study in a new place, where you have never been before, instead of on your couch where everything is familiar, you will create a much stronger memory of the material.”
The research team included postdoctoral fellow Jie Zheng, PhD, and neuroscientist Gabriel Kreiman, PhD, from Boston Children’s Hospital; neurosurgeon Taufik A. Valiante, MD, PhD, of the University of Toronto; and Adam Mamelak, MD, professor of Neurosurgery and director of the Functional Neurosurgery Program at Cedars-Sinai.
In follow-up studies, the team plans to test the theory that boundary and event cells activate dopamine neurons when they fire, and that dopamine, a chemical that sends messages between cells, might be used as a therapy to strengthen memory formation.
Rutishauser and his team also noted during this study that when event cells fired in time with one of the brain’s internal rhythms, the theta rhythm—a repetitive pattern of activity linked to learning, memory and navigation—subjects were better able to remember the order of images they had seen. This is an important new insight because it shows that deep brain stimulation that adjusts theta rhythms could prove therapeutic for memory disorders.
“Theta rhythms are thought to be the ‘temporal glue’ for episodic memory,” said Zheng, first author of the study. “We think that firing of event cells in synchrony with the theta rhythm builds time-based links across different memory folders.”
For more on this research, see Researchers Discover How the Human Brain Separates, Stores, and Retrieves Memories.
Reference: “Neurons detect cognitive boundaries to structure episodic memories in humans” by Jie Zheng, Andrea G. P. Schjetnan, Mar Yebra, Bernard A. Gomes, Clayton P. Mosher, Suneil K. Kalia, Taufik A. Valiante, Adam N. Mamelak, Gabriel Kreiman and Ueli Rutishauser, 7 March 2022, Nature Neuroscience.
DOI: 10.1038/s41593-022-01020-w
The study was funded by National Institutes of Health Grants number U01NS103792 and U01NS117839, National Science Foundation Grant number 8241231216, and Brain Canada.
MIT Researchers Create a Tool for Predicting the Future
Researchers design a user-friendly interface that helps nonexperts make forecasts using data collected over time.
Whether someone is trying to predict tomorrow’s weather, forecast future stock prices, identify missed opportunities for sales in retail, or estimate a patient’s risk of developing a disease, they will likely need to interpret time-series data, which are a collection of observations recorded over time.
Making predictions using time-series data typically requires several data-processing steps and the use of complex machine-learning algorithms, which have such a steep learning curve they aren’t readily accessible to nonexperts.
To make these powerful tools more user-friendly, MIT researchers developed a system that directly integrates prediction functionality on top of an existing time-series database. Their simplified interface, which they call tspDB (time series predict database), does all the complex modeling behind the scenes so a nonexpert can easily generate a prediction in only a few seconds.
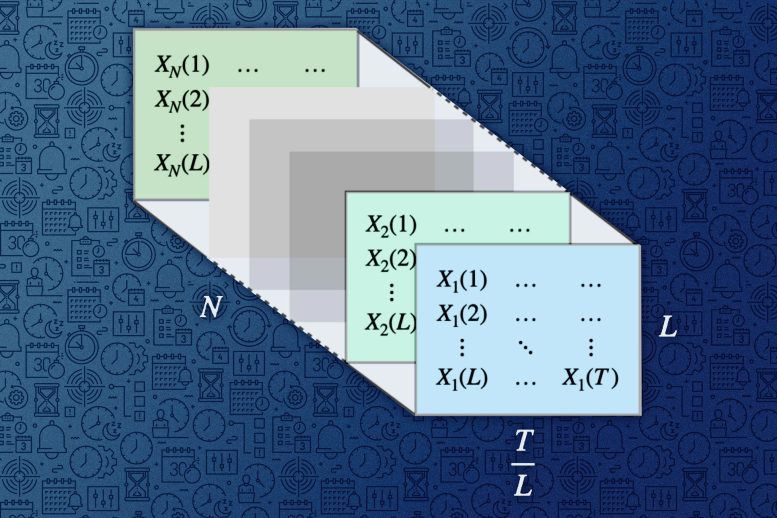
MIT researchers created a tool that enables people to make highly accurate predictions using multiple time-series data with just a few keystrokes. The powerful algorithm at the heart of their tool can transform multiple time series into a tensor, which is a multi-dimensional array of numbers (pictured). Credit: Figure courtesy of the researchers and edited by MIT News
The new system is more accurate and more efficient than state-of-the-art deep learning methods when performing two tasks: predicting future values and filling in missing data points.
One reason tspDB is so successful is that it incorporates a novel time-series-prediction algorithm, explains electrical engineering and computer science (EECS) graduate student Abdullah Alomar, an author of a recent research paper in which he and his co-authors describe the algorithm. This algorithm is especially effective at making predictions on multivariate time-series data, which are data that have more than one time-dependent variable. In a weather database, for instance, temperature, dew point, and cloud cover each depend on their past values.
The algorithm also estimates the volatility of a multivariate time series to provide the user with a confidence level for its predictions.
“Even as the time-series data becomes more and more complex, this algorithm can effectively capture any time-series structure out there. It feels like we have found the right lens to look at the model complexity of time-series data,” says senior author Devavrat Shah, the Andrew and Erna Viterbi Professor in EECS and a member of the Institute for Data, Systems, and Society and of the Laboratory for Information and Decision Systems.
Joining Alomar and Shah on the paper is lead author Anish Agrawal, a former EECS graduate student who is currently a postdoc at the Simons Institute at the University of California at Berkeley. The research will be presented at the ACM SIGMETRICS conference.
Adapting a new algorithm
Shah and his collaborators have been working on the problem of interpreting time-series data for years, adapting different algorithms and integrating them into tspDB as they built the interface.
About four years ago, they learned about a particularly powerful classical algorithm, called singular spectrum analysis (SSA), that imputes and forecasts single time series. Imputation is the process of replacing missing values or correcting past values. While this algorithm required manual parameter selection, the researchers suspected it could enable their interface to make effective predictions using time series data. In earlier work, they removed this need to manually intervene for algorithmic implementation.
The algorithm for single time series transformed it into a matrix and utilized matrix estimation procedures. The key intellectual challenge was how to adapt it to utilize multiple time series. After a few years of struggle, they realized the answer was something very simple: “Stack” the matrices for each individual time series, treat it as a one big matrix, and then apply the single time-series algorithm on it.
This utilizes information across multiple time series naturally — both across the time series and across time, which they describe in their new paper.
This recent publication also discusses interesting alternatives, where instead of transforming the multivariate time series into a big matrix, it is viewed as a three-dimensional tensor. A tensor is a multi-dimensional array, or grid, of numbers. This established a promising connection between the classical field of time series analysis and the growing field of tensor estimation, Alomar says.
“The variant of mSSA that we introduced actually captures all of that beautifully. So, not only does it provide the most likely estimation, but a time-varying confidence interval, as well,” Shah says.
The simpler, the better
They tested the adapted mSSA against other state-of-the-art algorithms, including deep-learning methods, on real-world time-series datasets with inputs drawn from the electricity grid, traffic patterns, and financial markets.
Their algorithm outperformed all the others on imputation and it outperformed all but one of the other algorithms when it came to forecasting future values. The researchers also demonstrated that their tweaked version of mSSA can be applied to any kind of time-series data.
“One reason I think this works so well is that the model captures a lot of time series dynamics, but at the end of the day, it is still a simple model. When you are working with something simple like this, instead of a neural network that can easily overfit the data, you can actually perform better,” Alomar says.
The impressive performance of mSSA is what makes tspDB so effective, Shah explains. Now, their goal is to make this algorithm accessible to everyone.
One a user installs tspDB on top of an existing database, they can run a prediction query with just a few keystrokes in about 0.9 milliseconds, as compared to 0.5 milliseconds for a standard search query. The confidence intervals are also designed to help nonexperts to make a more informed decision by incorporating the degree of uncertainty of the predictions into their decision making.
For instance, the system could enable a nonexpert to predict future stock prices with high accuracy in just a few minutes, even if the time-series dataset contains missing values.
Now that the researchers have shown why mSSA works so well, they are targeting new algorithms that can be incorporated into tspDB. One of these algorithms utilizes the same model to automatically enable change point detection, so if the user believes their time series will change its behavior at some point, the system will automatically detect that change and incorporate that into its predictions.
They also want to continue gathering feedback from current tspDB users to see how they can improve the system’s functionality and user-friendliness, Shah says.
“Our interest at the highest level is to make tspDB a success in the form of a broadly utilizable, open-source system. Time-series data are very important, and this is a beautiful concept of actually building prediction functionalities directly into the database. It has never been done before, and so we want to make sure the world uses it,” he says.
“This work is very interesting for a number of reasons. It provides a practical variant of mSSA which requires no hand tuning, they provide the first known analysis of mSSA, and the authors demonstrate the real-world value of their algorithm by being competitive with or out-performing several known algorithms for imputations and predictions in (multivariate) time series for several real-world data sets,” says Vishal Misra, a professor of computer science at Columbia University who was not involved with this research. “At the heart of it all is the beautiful modeling work where they cleverly exploit correlations across time (within a time series) and space (across time series) to create a low-rank spatiotemporal factor representation of a multivariate time series. Importantly this model connects the field of time series analysis to that of the rapidly evolving topic of tensor completion, and I expect a lot of follow-on research spurred by this paper.”
Reference: “On Multivariate Singular Spectrum Analysis and its Variants” by Anish Agarwal, Abdullah Alomar and Devavrat Shah, 13 February 2021, Computer Science > Machine Learning.
arXiv:2006.13448
No comments:
Post a Comment