Exploring the self-organizing origins of life
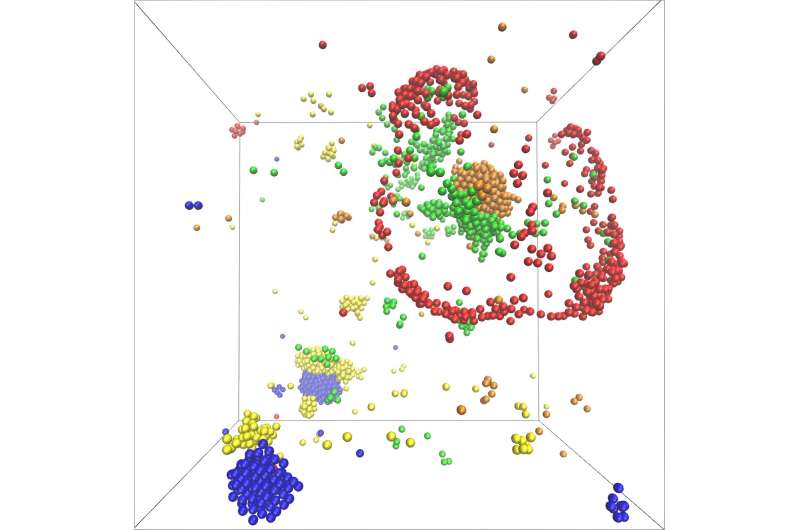
Catalytic molecules can form metabolically active clusters by creating and following concentration gradients—this is the result of a new study by scientists from the Max Planck Institute for Dynamics and Self-Organization (MPI-DS). Their model predicts the self-organization of molecules involved in metabolic pathways, adding a possible new mechanism to the theory of the origin of life.
The results can help to better understand how molecules participating in complex biological networks can form dynamic functional structures, and provide a platform for experiments on the origins of life.
One possible scenario for the origin of life is the spontaneous organization of interacting molecules into cell-like droplets. These molecular species would form the first self-replicating metabolic cycles, which are ubiquitous in biology and common throughout all organisms. According to this paradigm, the first biomolecules would need to cluster together through slow and overall inefficient processes.
Such slow cluster formation seems incompatible with how quickly life has appeared. Scientists from the department of Living Matter Physics from MPI-DS have now proposed an alternative model that explains such cluster formation and thus the fast onset of the chemical reactions required to form life.
"For this, we considered different molecules, in a simple metabolic cycle, where each species produces a chemical used by the next one," says Vincent Ouazan-Reboul, the first author of the study. "The only elements in the model are the catalytic activity of the molecules, their ability to follow concentration gradients of the chemicals they produce and consume, as well as the information on the order of molecules in the cycle," he continues.
Consequently, the model showed the formation of catalytic clusters including various molecular species. Furthermore, the growth of clusters happens exponentially fast. Molecules hence can assemble very quickly and in large numbers into dynamic structures.
"In addition, the number of molecule species which participate in the metabolic cycle plays a key role in the structure of the formed clusters," Ramin Golestanian, director at MPI-DS, summarizes, "Our model leads to a plethora of complex scenarios for self-organization and makes specific predictions about functional advantages that arise for odd or even number of participating species. It is remarkable that non-reciprocal interactions as required for our newly proposed scenario are generically present in all metabolic cycles."
In another study, the authors found that self-attraction is not required for clustering in a small metabolic network. Instead, network effects can cause even self-repelling catalysts to aggregate. With this, the researchers demonstrate new conditions in which complex interactions can create self-organized structures.
Overall, the new insights of both studies add another mechanism to the theory of how complex life once emerged from simple molecules, and more generally uncover how catalysts involved in metabolic networks can form structures.
The paper is published in the journal Nature Communications.
More information: Vincent Ouazan-Reboul et al, Self-organization of primitive metabolic cycles due to non-reciprocal interactions, Nature Communications (2023). DOI: 10.1038/s41467-023-40241-w
Journal information: Nature Communications
Provided by Max Planck Society
Mathematical theory predicts self-organized learning in real neurons
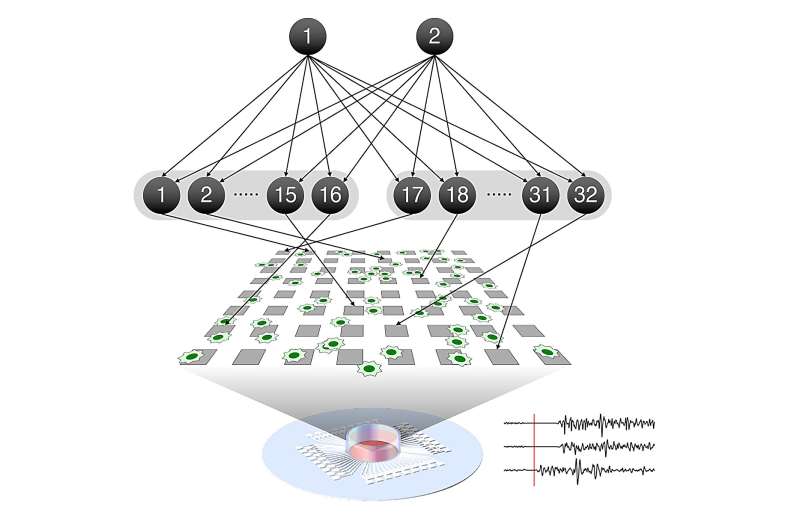
An international collaboration between researchers at the RIKEN Center for Brain Science (CBS) in Japan, the University of Tokyo, and University College London has demonstrated that self-organization of neurons as they learn follows a mathematical theory called the free energy principle.
The principle accurately predicted how real neural networks spontaneously reorganize to distinguish incoming information, as well as how altering neural excitability can disrupt the process. The findings thus have implications for building animal-like artificial intelligences and for understanding cases of impaired learning. The study was published August 7 in Nature Communications.
When we learn to tell the difference between voices, faces, or smells, networks of neurons in our brains automatically organize themselves so that they can distinguish between the different sources of incoming information. This process involves changing the strength of connections between neurons, and is the basis of all learning in the brain.
Takuya Isomura from RIKEN CBS and his international colleagues recently predicted that this type of network self-organization follows the mathematical rules that define the free energy principle. In the new study, they put this hypothesis to the test in neurons taken from the brains of rat embryos and grown in a culture dish on top of a grid of tiny electrodes.
Once you can distinguish two sensations, like voices, you will find that some of your neurons respond to one of the voices, while other neurons respond to the other voice. This is the result of neural network reorganization, which we call learning. In their culture experiment, the researchers mimicked this process by using the grid of electrodes beneath the neural network to stimulate the neurons in a specific pattern that mixed two separate hidden sources.
After 100 training sessions, the neurons automatically became selective—some responding very strongly to source #1 and very weakly to source #2, and others responding in the reverse. Drugs that either raise or lower neuron excitability disrupted the learning process when added to the culture beforehand. This shows that the cultured neurons do just what neurons are thought to do in the working brain.
The free energy principle states that this type of self-organization will follow a pattern that always minimizes the free energy in the system. To determine whether this principle is the guiding force behind neural network learning, the team used the real neural data to reverse engineer a predictive model based on it. Then, they fed the data from the first 10 electrode training sessions into the model and used it to make predictions about the next 90 sessions.
At each step, the model accurately predicted the responses of neurons and the strength of connectivity between neurons. This means that simply knowing the initial state of the neurons is enough to determine how the network would change over time as learning occurred.
"Our results suggest that the free-energy principle is the self-organizing principle of biological neural networks," says Isomura. "It predicted how learning occurred upon receiving particular sensory inputs and how it was disrupted by alterations in network excitability induced by drugs."
"Although it will take some time, ultimately, our technique will allow modeling the circuit mechanisms of psychiatric disorders and the effects of drugs such as anxiolytics and psychedelics," says Isomura. "Generic mechanisms for acquiring the predictive models can also be used to create next-generation artificial intelligences that learn as real neural networks do."
More information: Nature Communications (2023). DOI: 10.1038/s41467-023-40141-z