A model that can help inexperienced users identify phishing emails
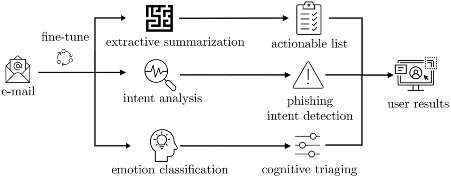
Phishing attacks are cyber-attacks through which criminals trick users into sending them money and sensitive information, or into installing malware on their computer, by sending them deceptive emails or messages. As these attacks have become increasingly widespread, developers have been trying to develop more advanced tools to detect them and protect potential victims.
Researchers at Monash University and CSIRO's Data61 in Australia have recently developed a machine learning-based approach that could help users to identify phishing emails, so that they don't inadvertently install malware or send sensitive data to cyber-criminals. This model was introduced in a paper pre-published on arXiv and set to be presented at AsiaCCS 2022, a cyber-security conference.
"We have identified a gap in current phishing research, namely realizing that existing literature focuses on rigorous 'black and white' methods to classify whether something is a phishing email or not," Tingmin (Tina) Wu, one of the researchers who carried out the study, told TechXplore.
Researchers have recently tried to develop models that can automatically analyze emails in people's inbox and detect phishing messages. Most of these methods, however, were found to only identify a limited number of patterns, thus missing many malicious emails.
"In contrast with other 'black and white' methods, we hand the power to decide whether something is suspicious over to the users, by equipping them with easily understandable machine results and conversions," Wu explained. "The reasoning behind this is that recent phishing attacks might not have obvious malicious patterns but instead can leverage human psychology to persuade users to hand over their personal information."
After realizing that automated phishing email detection methods did not achieve satisfactory results, researchers started shifting their focus on the introduction of detection support tools, such as security warnings, which allow users to make the final decision about whether to delete emails or not. These warnings, however, also proved to be ineffective, as they can be too technical for non-expert users.
Wu and her colleagues thus set out to develop an alternative tool for helping non-expert email users to determine what emails are safe and which are potentially malicious. The model they developed was designed to produce a more "digestible" summary of emails, which highlights emotional triggers, key content of the text, and the result of an intent analysis
"Our system summarizes phishing emails from three different angles to users to make informed decisions," Wu said. "Firstly, we summarize the emails using a variety of machine learning models to create an accurate, short summary so that users can quickly be aware of the most important content in the email."
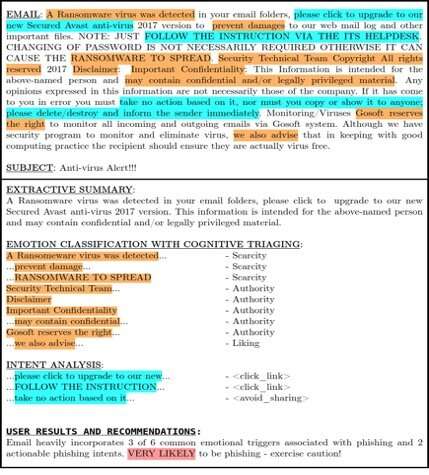
After it creates a digestible summary of the content of emails, the tool developed by Wu and her colleagues tries to identify the possible intent of phishing emails, so that users can make more informed decisions about what to do with the email. For instance, it shows them if an email from an unknown contact is asking them to click on a link. Finally, the approach created by the researchers also tries to identify emotional triggers.
"We derive a model to extract the cognitive triggers based on the language used in the emails," Wu said. "One example of a psychological weakness used by attackers is that users might tend to obey the request when it comes to punishment if not complying with it. The information from these three branches is merged to support users to make the final decision."
Instead of automatically detecting and filtering potentially malicious emails, the approach devised by Wu and her colleagues prepares a summary of emails that users can then use to decide what to do with different emails in their inbox. By using the tool regularly, therefore, non-expert users can learn to identify common patterns in phishing by themselves.
The model introduced by the researchers combines a variety of state-of-the-art phishing detection methods into a single, concise "informational package." In contrast with other previously proposed approaches, therefore, it presents users with probabilities, instead of "hard truths," preventing errors that might result in the loss of important messages.
"Our system is designed to address the challenges of improving the readability and effectiveness of generated information on phishing emails," Wu said. "While most of the current warnings are generated based on the URL, our method focuses on generating useful information around the intention of the emails. That is, to help users identify the phishing attempts by better leveraging their contextual knowledge and aim at the latest trending tactics, e.g., using phishing emails that can easily bypass URL-based detection."
The recent work by this team of researchers introduces an alternative approach for decreasing the impact of phishing attack, which does not rely on error-prone automated systems or on pop-up windows that users typically ignore. So far, the team created an elementary proof-of-concept of their system, but they now plan to develop it further.
"We now plan to continue improving our system," Wu added. "We will keep collecting the new datasets and make sure the model can extract the useful contents from the emails no matter how the attacking tactic evolves. We will also conduct a large-scale user study to ensure the system is user-friendly and effective."
In the future, the system developed by Wu and her colleagues could open new possibilities for tackling phishing attacks. In addition, it could help email providers to teach non-expert users to independently detect these malicious messages, thus potentially reducing their impact.
"Human-centric systems are the first step toward leveraging the complementary intelligence of humans and machines," Wu added. "Some future studies are still needed, e.g., to investigate the impact of the human factors on the final decision, to understand users' habituation in long-time interacting with the warnings and implementing the system in a broad area in cybersecurity, not only phishing."A new model to automatically detect and filter spam emails
More information: Amir Kashapov, Tingmin Wu, Alsharif Abuadbba, Carsten Rudolph, Email summarization to assist users in phishing identification. arXiv:2203.13380v1 [cs.CR], arxiv.org/abs/2203.13380
© 2022 Science X Network
No comments:
Post a Comment