If AI is going to help us in a crisis, we need a new kind of ethics
Ethics for urgency means making ethics a core part of AI rather than an afterthought, says Jess Whittlestone.
by Will Douglas Heaven June 24, 2020

MS TECH | PIXABAY
Jess Whittlestone at the Leverhulme Centre for the Future of Intelligence at the University of Cambridge and her colleagues published a comment piece in Nature Machine Intelligence this week arguing that if artificial intelligence is going to help in a crisis, we need a new, faster way of doing AI ethics, which they call ethics for urgency.
Jess Whittlestone at the Leverhulme Centre for the Future of Intelligence at the University of Cambridge and her colleagues published a comment piece in Nature Machine Intelligence this week arguing that if artificial intelligence is going to help in a crisis, we need a new, faster way of doing AI ethics, which they call ethics for urgency.
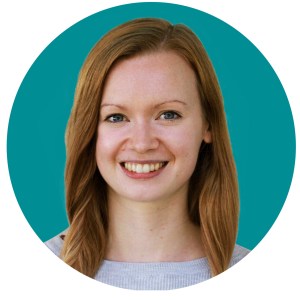
JESS WHITTLESTONE
For Whittlestone, this means anticipating problems before they happen, finding better ways to build safety and reliability into AI systems, and emphasizing technical expertise at all levels of the technology’s development and use. At the core of these recommendations is the idea that ethics needs to become simply a part of how AI is made and used, rather than an add-on or afterthought.
Ultimately, AI will be quicker to deploy when needed if it is made with ethics built in, she argues. I asked her to talk me through what this means.
This interview has been edited for length and clarity.
Why do we need a new kind of ethics for AI?
With this pandemic we’re suddenly in a situation where people are really talking about whether AI could be useful, whether it could save lives. But the crisis has made it clear that we don’t have robust enough ethics procedures for AI to be deployed safely, and certainly not ones that can be implemented quickly.
What’s wrong with the ethics we have?
I spent the last couple of years reviewing AI ethics initiatives, looking at their limitations and asking what else we need. Compared to something like biomedical ethics, the ethics we have for AI isn’t very practical. It focuses too much on high-level principles. We can all agree that AI should be used for good. But what does that really mean? And what happens when high-level principles come into conflict?
For example, AI has the potential to save lives but this could come at the cost of civil liberties like privacy. How do we address those trade-offs in ways that are acceptable to lots of different people? We haven’t figured out how to deal with the inevitable disagreements.
AI ethics also tends to respond to existing problems rather than anticipate new ones. Most of the issues that people are discussing today around algorithmic bias came up only when high-profile things went wrong, such as with policing and parole decisions.
But ethics needs to be proactive and prepare for what could go wrong, not what has gone wrong already. Obviously, we can’t predict the future. But as these systems become more powerful and get used in more high-stakes domains, the risks will get bigger.
What opportunities have we missed by not having these procedures in place?
It’s easy to overhype what’s possible, and AI was probably never going to play a huge role in this crisis. Machine-learning systems are not mature enough.
But there are a handful of cases in which AI is being tested for medical diagnosis or for resource allocation across hospitals. We might have been able to use those sorts of systems more widely, reducing some of the load on health care, had they been designed from the start with ethics in mind.
With resource allocation in particular, you are deciding which patients are highest priority. You need an ethical framework built in before you use AI to help with those kinds of decisions.
So is ethics for urgency simply a call to make existing AI ethics better?
That’s part of it. The fact that we don’t have robust, practical processes for AI ethics makes things more difficult in a crisis scenario. But in times like this you also have greater need for transparency. People talk a lot about the lack of transparency with machine-learning systems as black boxes. But there is another kind of transparency, concerning how the systems are used.
This is especially important in a crisis, when governments and organizations are making urgent decisions that involve trade-offs. Whose health do you prioritize? How do you save lives without destroying the economy? If an AI is being used in public decision-making, transparency is more important than ever.
What needs to change?
We need to think about ethics differently. It shouldn’t be something that happens on the side or afterwards—something that slows you down. It should simply be part of how we build these systems in the first place: ethics by design.
I sometimes feel “ethics” is the wrong word. What we’re saying is that machine-learning researchers and engineers need to be trained to think through the implications of what they’re building, whether they’re doing fundamental research like designing a new reinforcement-learning algorithm or something more practical like developing a health-care application. If their work finds its way into real-world products and services, what might that look like? What kinds of issues might it raise?
Some of this has started already. We are working with some early-career AI researchers, talking to them about how to bring this way of thinking to their work. It’s a bit of an experiment, to see what happens. But even NeurIPS [a leading AI conference] now asks researchers to include a statement at the end of their papers outlining potential societal impacts of their work.
You’ve said that we need people with technical expertise at all levels of AI design and use. Why is that?
I’m not saying that technical expertise is the be-all and end-all of ethics, but it’s a perspective that needs to be represented. And I don’t want to sound like I’m saying all the responsibility is on researchers, because a lot of the important decisions about how AI gets used are made further up the chain, by industry or by governments.
But I worry that the people who are making those decisions don’t always fully understand the ways it might go wrong. So you need to involve people with technical expertise. Our intuitions about what AI can and can’t do are not very reliable.
What you need at all levels of AI development are people who really understand the details of machine learning to work with people who really understand ethics. Interdisciplinary collaboration is hard, however. People with different areas of expertise often talk about things in different ways. What a machine-learning researcher means by privacy may be very different from what a lawyer means by privacy, and you can end up with people talking past each other. That’s why it’s important for these different groups to get used to working together.
You’re pushing for a pretty big institutional and cultural overhaul. What makes you think people will want to do this rather than set up ethics boards or oversight committees—which always make me sigh a bit because they tend to be toothless?
Yeah, I also sigh. But I think this crisis is forcing people to see the importance of practical solutions. Maybe instead of saying, “Oh, let’s have this oversight board and that oversight board,” people will be saying, “We need to get this done, and we need to get it done properly.”
WHOSE ETHICS
No comments:
Post a Comment