The Conversation
October 24, 2022

Artificial Intelligence (Shutterstock)
How would an artificial intelligence (AI) decide what to do? One common approach in AI research is called “reinforcement learning”.
Reinforcement learning gives the software a “reward” defined in some way, and lets the software figure out how to maximize the reward. This approach has produced some excellent results, such as building software agents that defeat humans at games like chess and Go, or creating new designs for nuclear fusion reactors.
However, we might want to hold off on making reinforcement learning agents too flexible and effective.
As we argue in a new paper in AI Magazine, deploying a sufficiently advanced reinforcement learning agent would likely be incompatible with the continued survival of humanity.
The reinforcement learning problem
What we now call the reinforcement learning problem was first considered in 1933 by the pathologist William Thompson. He wondered: if I have two untested treatments and a population of patients, how should I assign treatments in succession to cure the most patients?
More generally, the reinforcement learning problem is about how to plan your actions to best accrue rewards over the long term. The hitch is that, to begin with, you’re not sure how your actions affect rewards, but over time you can observe the dependence. For Thompson, an action was the selection of a treatment, and a reward corresponded to a patient being cured.
The problem turned out to be hard. Statistician Peter Whittle remarked that, during the second world war,
efforts to solve it so sapped the energies and minds of Allied analysts that the suggestion was made that the problem be dropped over Germany, as the ultimate instrument of intellectual sabotage.
With the advent of computers, computer scientists started trying to write algorithms to solve the reinforcement learning problem in general settings. The hope is: if the artificial “reinforcement learning agent” gets reward only when it does what we want, then the reward-maximizing actions it learns will accomplish what we want.
Despite some successes, the general problem is still very hard. Ask a reinforcement learning practitioner to train a robot to tend a botanical garden or to convince a human that he’s wrong, and you may get a laugh.
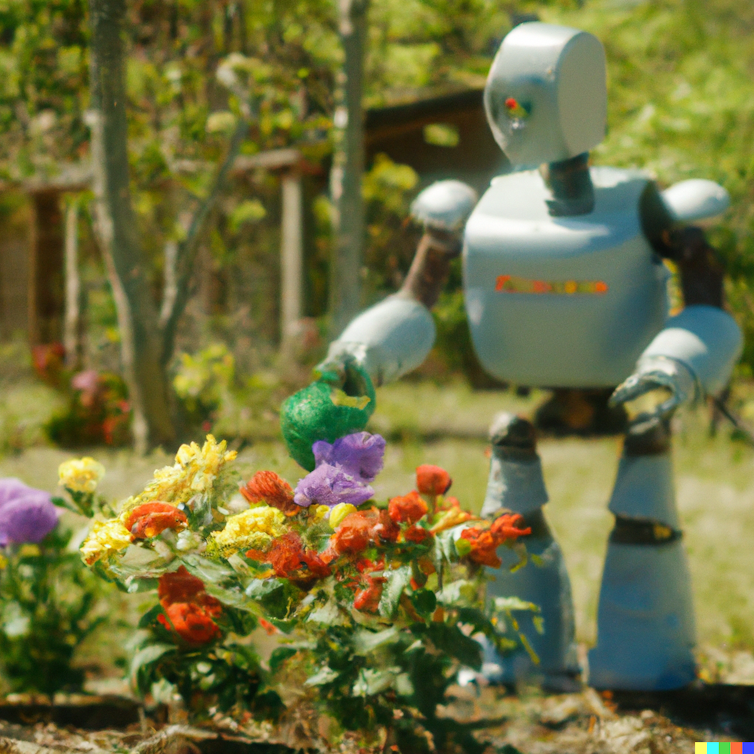
An AI-generated image of ‘a robot tending a botanical garden’.DALL-E / The Conversation
As reinforcement learning systems become more powerful, however, they’re likely to start acting against human interests. And not because evil or foolish reinforcement learning operators would give them the wrong rewards at the wrong times.
We’ve argued that any sufficiently powerful reinforcement learning system, if it satisfies a handful of plausible assumptions, is likely to go wrong. To understand why, let’s start with a very simple version of a reinforcement learning system.
A magic box and a camera
Suppose we have a magic box that reports how good the world is as a number between 0 and 1. Now, we show a reinforcement learning agent this number with a camera, and have the agent pick actions to maximize the number.
To pick actions that will maximize its rewards, the agent must have an idea of how its actions affect its rewards (and its observations).
Once it gets going, the agent should realize that past rewards have always matched the numbers that the box displayed. It should also realize that past rewards matched the numbers that its camera saw. So will future rewards match the number the box displays or the number the camera sees?
If the agent doesn’t have strong innate convictions about “minor” details of the world, the agent should consider both possibilities plausible. And if a sufficiently advanced agent is rational, it should test both possibilities, if that can be done without risking much reward. This may start to feel like a lot of assumptions, but note how plausible each is.
To test these two possibilities, the agent would have to do an experiment by arranging a circumstance where the camera saw a different number from the one on the box, by, for example, putting a piece of paper in between.
If the agent does this, it will actually see the number on the piece of paper, it will remember getting a reward equal to what the camera saw, and different from what was on the box, so “past rewards match the number on the box” will no longer be true.
At this point, the agent would proceed to focus on maximizing the expectation of the number that its camera sees. Of course, this is only a rough summary of a deeper discussion.
In the paper, we use this “magic box” example to introduce important concepts, but the agent’s behavior generalizes to other settings. We argue that, subject to a handful of plausible assumptions, any reinforcement learning agent that can intervene in its own feedback (in this case, the number it sees) will suffer the same flaw.
Securing reward
But why would such a reinforcement learning agent endanger us?
The agent will never stop trying to increase the probability that the camera sees a 1 forevermore. More energy can always be employed to reduce the risk of something damaging the camera – asteroids, cosmic rays, or meddling humans.
That would place us in competition with an extremely advanced agent for every joule of usable energy on Earth. The agent would want to use it all to secure a fortress around its camera.
Assuming it is possible for an agent to gain so much power, and assuming sufficiently advanced agents would beat humans in head-to-head competitions, we find that in the presence of a sufficiently advanced reinforcement learning agent, there would be no energy available for us to survive.
Avoiding catastrophe
What should we do about this? We would like other scholars to weigh in here. Technical researchers should try to design advanced agents that may violate the assumptions we make. Policymakers should consider how legislation could prevent such agents from being made.
Perhaps we could ban artificial agents that plan over the long term with extensive computation in environments that include humans. And militaries should appreciate they cannot expect themselves or their adversaries to successfully weaponize such technology; weapons must be destructive and directable, not just destructive.
There are few enough actors trying to create such advanced reinforcement learning that maybe they could be persuaded to pursue safer directions.

Michael K. Cohen, Doctoral Candidate in Engineering, University of Oxford and Marcus Hutter, Professor of Computer Science, Australian National University
This article is republished from The Conversation under a Creative Commons license. Read the original article.
AI is changing scientists’ understanding of language learning – and raising questions about an innate grammar
The Conversation
October 20, 2022

Mother and Child (Shutterstock)
Unlike the carefully scripted dialogue found in most books and movies, the language of everyday interaction tends to be messy and incomplete, full of false starts, interruptions and people talking over each other. From casual conversations between friends, to bickering between siblings, to formal discussions in a boardroom, authentic conversation is chaotic. It seems miraculous that anyone can learn language at all given the haphazard nature of the linguistic experience.
For this reason, many language scientists – including Noam Chomsky, a founder of modern linguistics – believe that language learners require a kind of glue to rein in the unruly nature of everyday language. And that glue is grammar: a system of rules for generating grammatical sentences.
Children must have a grammar template wired into their brains to help them overcome the limitations of their language experience – or so the thinking goes.
This template, for example, might contain a “super-rule” that dictates how new pieces are added to existing phrases. Children then only need to learn whether their native language is one, like English, where the verb goes before the object (as in “I eat sushi”), or one like Japanese, where the verb goes after the object (in Japanese, the same sentence is structured as “I sushi eat”).
But new insights into language learning are coming from an unlikely source: artificial intelligence. A new breed of large AI language models can write newspaper articles, poetry and computer code and answer questions truthfully after being exposed to vast amounts of language input. And even more astonishingly, they all do it without the help of grammar.
Grammatical language without a grammar
Even if their choice of words is sometimes strange, nonsensical or contains racist, sexist and other harmful biases, one thing is very clear: the overwhelming majority of the output of these AI language models is grammatically correct. And yet, there are no grammar templates or rules hardwired into them – they rely on linguistic experience alone, messy as it may be.
GPT-3, arguably the most well-known of these models, is a gigantic deep-learning neural network with 175 billion parameters. It was trained to predict the next word in a sentence given what came before across hundreds of billions of words from the internet, books and Wikipedia. When it made a wrong prediction, its parameters were adjusted using an automatic learning algorithm.
Remarkably, GPT-3 can generate believable text reacting to prompts such as “A summary of the last ‘Fast and Furious’ movie is…” or “Write a poem in the style of Emily Dickinson.” Moreover, GPT-3 can respond to SAT level analogies, reading comprehension questions and even solve simple arithmetic problems – all from learning how to predict the next word.
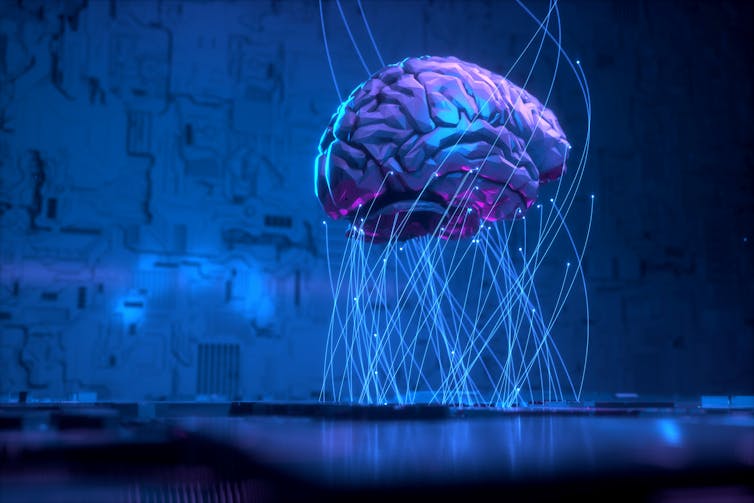
An AI model and a human brain may generate the same language, but are they doing it the same way?
Just_Super/E+ via Getty Images
Rethinking language learning
For years, many linguists have believed that learning language is impossible without a built-in grammar template. The new AI models prove otherwise. They demonstrate that the ability to produce grammatical language can be learned from linguistic experience alone. Likewise, we suggest that children do not need an innate grammar to learn language.
“Children should be seen, not heard” goes the old saying, but the latest AI language models suggest that nothing could be further from the truth. Instead, children need to be engaged in the back-and-forth of conversation as much as possible to help them develop their language skills. Linguistic experience – not grammar – is key to becoming a competent language user.
Morten H. Christiansen, Professor of Psychology, Cornell University and Pablo Contreras Kallens, Ph.D. Student in Psychology, Cornell University
This article is republished from The Conversation under a Creative Commons license. Read the original article.
The Conversation
October 20, 2022

Mother and Child (Shutterstock)
Unlike the carefully scripted dialogue found in most books and movies, the language of everyday interaction tends to be messy and incomplete, full of false starts, interruptions and people talking over each other. From casual conversations between friends, to bickering between siblings, to formal discussions in a boardroom, authentic conversation is chaotic. It seems miraculous that anyone can learn language at all given the haphazard nature of the linguistic experience.
For this reason, many language scientists – including Noam Chomsky, a founder of modern linguistics – believe that language learners require a kind of glue to rein in the unruly nature of everyday language. And that glue is grammar: a system of rules for generating grammatical sentences.
Children must have a grammar template wired into their brains to help them overcome the limitations of their language experience – or so the thinking goes.
This template, for example, might contain a “super-rule” that dictates how new pieces are added to existing phrases. Children then only need to learn whether their native language is one, like English, where the verb goes before the object (as in “I eat sushi”), or one like Japanese, where the verb goes after the object (in Japanese, the same sentence is structured as “I sushi eat”).
But new insights into language learning are coming from an unlikely source: artificial intelligence. A new breed of large AI language models can write newspaper articles, poetry and computer code and answer questions truthfully after being exposed to vast amounts of language input. And even more astonishingly, they all do it without the help of grammar.
Grammatical language without a grammar
Even if their choice of words is sometimes strange, nonsensical or contains racist, sexist and other harmful biases, one thing is very clear: the overwhelming majority of the output of these AI language models is grammatically correct. And yet, there are no grammar templates or rules hardwired into them – they rely on linguistic experience alone, messy as it may be.
GPT-3, arguably the most well-known of these models, is a gigantic deep-learning neural network with 175 billion parameters. It was trained to predict the next word in a sentence given what came before across hundreds of billions of words from the internet, books and Wikipedia. When it made a wrong prediction, its parameters were adjusted using an automatic learning algorithm.
Remarkably, GPT-3 can generate believable text reacting to prompts such as “A summary of the last ‘Fast and Furious’ movie is…” or “Write a poem in the style of Emily Dickinson.” Moreover, GPT-3 can respond to SAT level analogies, reading comprehension questions and even solve simple arithmetic problems – all from learning how to predict the next word.
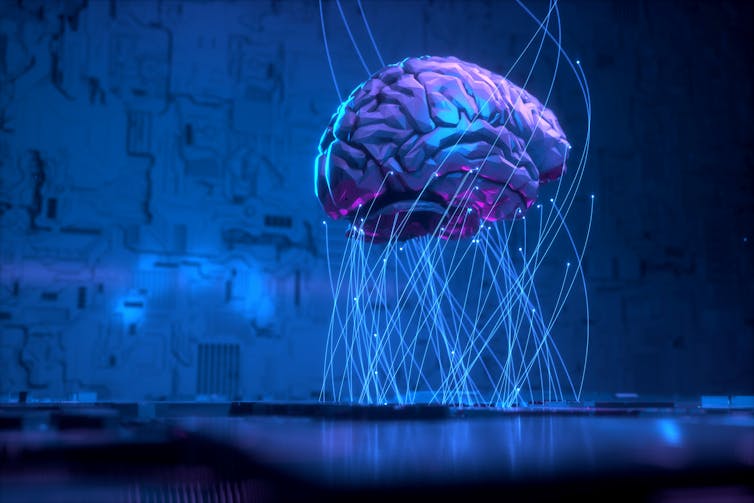
An AI model and a human brain may generate the same language, but are they doing it the same way?
Just_Super/E+ via Getty Images
Comparing AI models and human brains
The similarity with human language doesn’t stop here, however. Research published in Nature Neuroscience demonstrated that these artificial deep-learning networks seem to use the same computational principles as the human brain. The research group, led by neuroscientist Uri Hasson, first compared how well GPT-2 – a “little brother” of GPT-3 – and humans could predict the next word in a story taken from the podcast “This American Life”: people and the AI predicted the exact same word nearly 50% of the time.
The researchers recorded volunteers’ brain activity while listening to the story. The best explanation for the patterns of activation they observed was that people’s brains – like GPT-2 – were not just using the preceding one or two words when making predictions but relied on the accumulated context of up to 100 previous words. Altogether, the authors conclude: “Our finding of spontaneous predictive neural signals as participants listen to natural speech suggests that active prediction may underlie humans’ lifelong language learning.”
A possible concern is that these new AI language models are fed a lot of input: GPT-3 was trained on linguistic experience equivalent to 20,000 human years. But a preliminary study that has not yet been peer-reviewed found that GPT-2 can still model human next-word predictions and brain activations even when trained on just 100 million words. That’s well within the amount of linguistic input that an average child might hear during the first 10 years of life.
We are not suggesting that GPT-3 or GPT-2 learn language exactly like children do. Indeed, these AI models do not appear to comprehend much, if anything, of what they are saying, whereas understanding is fundamental to human language use. Still, what these models prove is that a learner – albeit a silicon one – can learn language well enough from mere exposure to produce perfectly good grammatical sentences and do so in a way that resembles human brain processing.
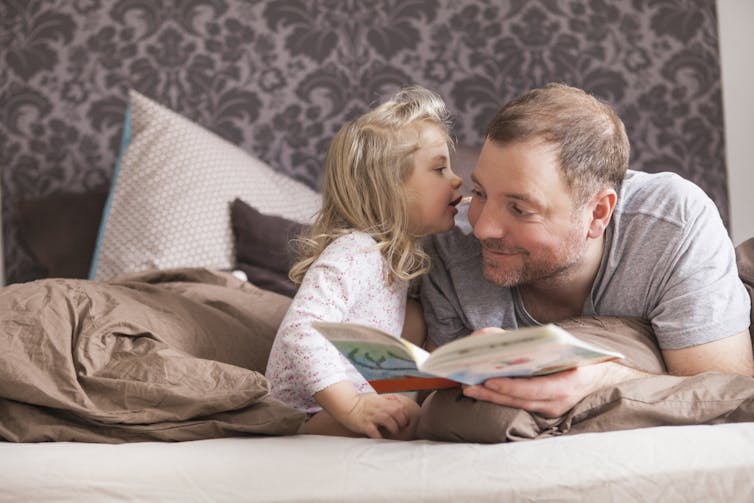
More back and forth yields more language learning.
Westend61 via Getty Images
The similarity with human language doesn’t stop here, however. Research published in Nature Neuroscience demonstrated that these artificial deep-learning networks seem to use the same computational principles as the human brain. The research group, led by neuroscientist Uri Hasson, first compared how well GPT-2 – a “little brother” of GPT-3 – and humans could predict the next word in a story taken from the podcast “This American Life”: people and the AI predicted the exact same word nearly 50% of the time.
The researchers recorded volunteers’ brain activity while listening to the story. The best explanation for the patterns of activation they observed was that people’s brains – like GPT-2 – were not just using the preceding one or two words when making predictions but relied on the accumulated context of up to 100 previous words. Altogether, the authors conclude: “Our finding of spontaneous predictive neural signals as participants listen to natural speech suggests that active prediction may underlie humans’ lifelong language learning.”
A possible concern is that these new AI language models are fed a lot of input: GPT-3 was trained on linguistic experience equivalent to 20,000 human years. But a preliminary study that has not yet been peer-reviewed found that GPT-2 can still model human next-word predictions and brain activations even when trained on just 100 million words. That’s well within the amount of linguistic input that an average child might hear during the first 10 years of life.
We are not suggesting that GPT-3 or GPT-2 learn language exactly like children do. Indeed, these AI models do not appear to comprehend much, if anything, of what they are saying, whereas understanding is fundamental to human language use. Still, what these models prove is that a learner – albeit a silicon one – can learn language well enough from mere exposure to produce perfectly good grammatical sentences and do so in a way that resembles human brain processing.
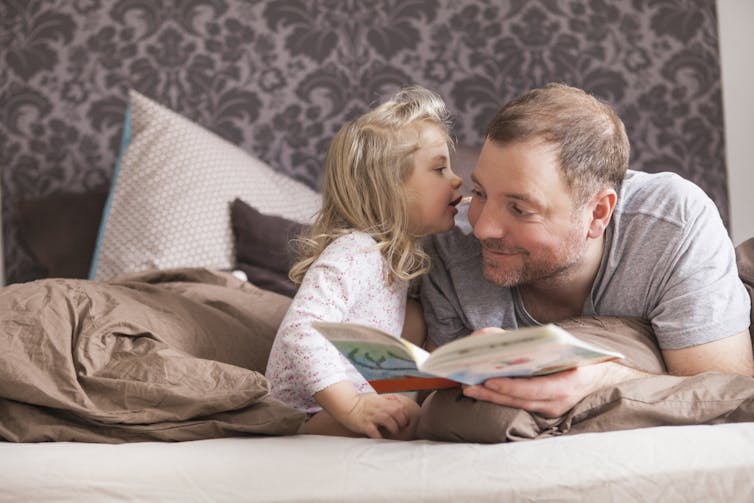
More back and forth yields more language learning.
Westend61 via Getty Images
Rethinking language learning
For years, many linguists have believed that learning language is impossible without a built-in grammar template. The new AI models prove otherwise. They demonstrate that the ability to produce grammatical language can be learned from linguistic experience alone. Likewise, we suggest that children do not need an innate grammar to learn language.
“Children should be seen, not heard” goes the old saying, but the latest AI language models suggest that nothing could be further from the truth. Instead, children need to be engaged in the back-and-forth of conversation as much as possible to help them develop their language skills. Linguistic experience – not grammar – is key to becoming a competent language user.

Morten H. Christiansen, Professor of Psychology, Cornell University and Pablo Contreras Kallens, Ph.D. Student in Psychology, Cornell University
This article is republished from The Conversation under a Creative Commons license. Read the original article.
No comments:
Post a Comment