
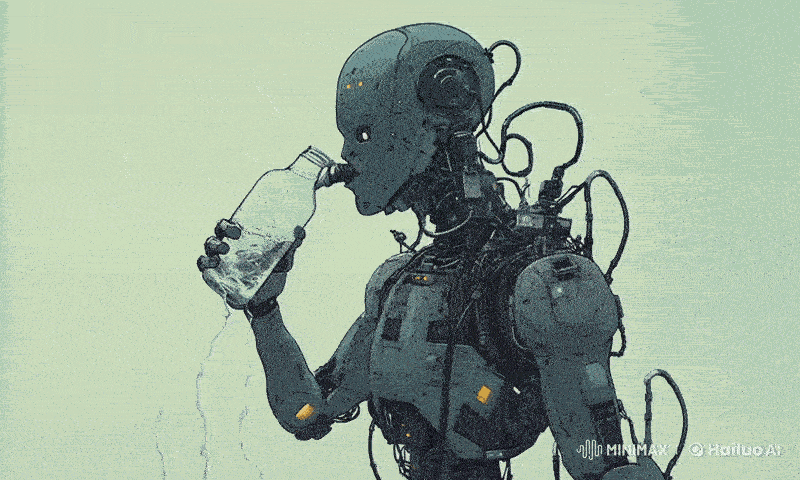
The climate paradox: AI’s role in both saving and sabotaging the planet
As the world hops on the AI bandwagon, one question continues to loom: is it sustainable?
December 10, 2024
PRISM/DAWN
In a world racing to combat climate change, the newest weapon in our arsenal comes with an ironic twist. Artificial Intelligence, our digital saviour in the fight against global warming, has developed quite a thirst of its own. In what could be a scene from a science fiction story, the very machines we’re building to help save our planet are guzzling water and energy at rates that have scientists very concerned.
Every time you ask ChatGPT — that marvel of modern AI that can write poems about climate change or calculate carbon footprints — a few dozen questions, it drinks roughly 500ml of clean water to cool the powerful computers behind its intelligence. That’s two glasses of water for a few dozen queries, while training a model like GPT-3, which is several generations behind OpenAI’s latest o1 model, consumes millions of litres more.
For Pakistan, perched precariously on the frontlines of the climate crisis, this paradox holds particular significance. In a country where glacial melt threatens northern communities and erratic monsoons flood southern plains, AI offers powerful tools for prediction and adaptation.
Yet as temperature records shatter each summer and water scarcity looms, the environmental cost of these digital solutions cannot be ignored. “Climate change is the biggest challenge facing the planet. It will need every solution possible, including technology like artificial intelligence,” notes Jackie Snow in National Geographic.
But as Sam Altman, OpenAI’s chief executive, recently admitted at the World Economic Forum, AI’s growing energy demands present their own challenge: “There’s no way to get there without a breakthrough.”

At its best, artificial intelligence acts like a planetary nervous system — sensing, predicting, and helping us respond to environmental threats. Google’s Flood Hub, for instance, provides flood forecasts up to seven days in advance across more than 80 countries, helping protect communities across Africa, Europe, South and Central America, and the Asia-Pacific region, including Pakistan. As of 2023, their forecasts cover areas where over 460 million people live. In October 2023, this service expanded to the US and Canada, covering more than 800 riverbanks where over 12m people live.
In California, machine learning algorithms help firefighters predict and track wildfire spread. And in agriculture, AI’s precision farming techniques are reducing water waste and optimising crop yields.
These applications aren’t just impressive — they’re essential. The World Meteorological Organisation estimates that improving early warning systems could reduce climate disaster damages by 30 per cent.
AI is already delivering real climate action results. Global Forest Watch uses AI and satellite imagery to create a real-time tool to monitor and combat deforestation. Google’s AI-powered project Green Light collaborates with 12 cities including Manchester, Rio de Janeiro, Jakarta, and Abu Dhabi to reduce stop-and-start traffic events through AI-supported traffic light management — with early indicators showing potential for up to a 30pc reduction in stops, which could reduce emissions at intersections by up to 10pc. In the fusion energy field, Google DeepMind has developed a deep reinforcement learning system that helps researchers better control nuclear fusion plasma, opening new pathways for clean energy research.
But running these powerful AI systems requires enormous computing power. For example, training GPT-3 consumed an estimated 1,287 MWh (megawatt-hours) of electricity, which is roughly equivalent to the energy consumption of an average Pakistani household over 2,000 years, if the 643 kWh average annual consumption figure is to be believed. This January, the International Energy Agency (IEA) forecast that global data centre electricity demand will more than double from 2022 to 2026, with AI fuelling that increase.
And their carbon footprint is equally large. Training a single large language model is equal to around 300,000kg of carbon dioxide emissions. This is of the order of 125 round-trip flights between New York and Beijing. According to researchers at the University of Massachusetts Amherst, training one of these models can generate carbon emissions equivalent to the lifetime emissions of five average American cars.
The water footprint is equally startling. Modern data centres use vast amounts of water for cooling — up to 500,000 gallons per day for a large facility. By 2027, some experts project that AI systems could demand as much water as half the United Kingdom’s annual consumption.

In west Des Moines, in America’s Iowa state, residents were startled to discover that a nearby data centre — one that helped train some of the world’s most advanced AI models, like GPT-4 — was consuming millions of gallons of their local water supply. A lawsuit by local residents revealed that in July 2022, the month before OpenAI finished training the model, the cluster used about 6pc of the district’s water.
“Within years, large AI systems are likely to need as much energy as entire nations,” warns Crawford. This isn’t hyperbole — the compute power needed for AI training has been doubling every 3.4 months since 2012, a staggering deviation from the traditional 18-month doubling of computer power known as Moore’s Law.
But it’s not just about keeping machines cool. Each component in an AI system’s lifecycle demands resources. Manufacturing the specialised chips that power AI requires ultra-pure water and rare earth minerals. The servers running these systems need constant power, and the batteries storing that power contain materials whose mining creates its own environmental challenges.

While the benefits of AI — improved climate predictions, optimised energy systems, better disaster responses — can be global, the environmental costs fall disproportionately on certain regions and communities.
The disparity is stark: in 2022, while Google operated its data centre in Finland on 97pc carbon-free energy, that number plummeted to between 4-18pc for its data centres in Asia. This dramatic difference means some communities bear a far heavier burden of fossil fuel consumption and air pollution than others. “Unfortunately, there remains a widening disparity in how different regions and communities are affected by AI’s environmental impacts,” note Shaolei Ren and Adam Wierman in the Harvard Business Review.
The scale of this challenge is set to grow dramatically — global AI energy demand is projected to increase tenfold, exceeding the annual electricity consumption of Belgium by 2026. In the United States alone, AI-driven data centre energy consumption is expected to reach about 6pc of the nation’s total electricity usage by that same year.
In water-stressed regions like Pakistan, where every drop counts, the water demands of AI infrastructure could compete with basic needs like agriculture and drinking water.
This disparity extends beyond resources to expertise. According to a Stack Overflow survey published by the OECD, North America hosts 30pc of the world’s AI experts (who are active online), while Pakistan accounts for 0.67pc. This concentration of talent and computing power in the Global North risks creating solutions that may not fully account for the needs and constraints of developing nations.

The path to sustainable AI isn’t just about accepting trade-offs; it’s about reimagining how we build and deploy these systems. Tech companies are making unprecedented investments in renewable energy. According to the latest Solar Means Business report, industry leaders including Amazon, Microsoft, Meta, and Google have signed renewable energy agreements totalling almost 50 GW of clean energy generation capacity — equivalent to the entire power generation capacity of Sweden.
But the real innovations are happening at the intersection of efficiency and design.
“Training better models can actually save more energy over time,” explains Justin Burr from Google AI. “Given the number of times they’re used each day for inference, in less than a week they save more in energy than the old hand-tuned versions.”
Google’s machine-learning optimised Tensor Processing Units (TPUs) demonstrate this principle in action — their version 4 can generate 93pc fewer emissions compared to traditional unoptimised servers.
Location matters too. The carbon intensity of AI training can vary dramatically depending on where the data centres are located. Norway’s electric grid, for instance, produces just 29g of CO2 per kilowatt-hour, compared to 709g in South Africa.
Some regions could turn geography into an advantage. In Quebec, for instance, the combination of hydroelectric power and cold winters creates a unique opportunity: data centres can tap into clean energy while their waste heat is repurposed to warm nearby homes.
“I think that more tax incentives should be given for cloud providers to open data centres in places with hydro or solar energy,” suggests Alexandra Luccioni, an AI researcher quoted in Nature. “In Quebec, we have a very low-carbon grid that relies mostly on hydro, plus with the cold winters, the heat generated by computing centres can be used to heat homes.”
The research community has also been pushing for what they call “Green AI” — a movement that prioritises computational efficiency alongside accuracy. Roy Schwartz and his colleagues found that 90pc of papers at top AI conferences prioritised accuracy over efficiency. But this is changing. Teams like France’s BigScience project have demonstrated that it’s possible to build models similar in size to GPT-3 with a much lower carbon footprint.
Water conservation is becoming a priority too. Meta’s data centres have experimented with raising the temperature and lowering humidity requirements, achieving water savings of up to 40pc in some cases. Google has gone further, pledging to replenish 120pc of the water it consumes by 2030 through initiatives including wetland restoration and rainwater harvesting.
The most promising approaches don’t just focus on individual metrics but take a holistic view of environmental impact. The Copenhagen Centre on Energy Efficiency emphasises that “environmental sustainability should be considered as one of the principles towards responsible development and application of AI”. This means considering everything from the sourcing of materials for AI hardware to the end-of-life disposal of equipment.
“Not every problem demands a machine learning-based solution,” notes Deepika Sandeep, an AI scientist who heads the AI and ML programme at Bharat Light & Power, a clean energy generation company based in Bangalore, India. He advocates for a judicious approach where simpler, less compute-intensive solutions are used when possible.
These improvements can’t come soon enough. But Bill Gates takes a more optimistic view of AI’s environmental equation. While concerns about AI’s energy appetite grow, Gates argues that the technology’s overall impact on climate change will be net positive. The additional strain that AI data centres put on power grids is relatively modest, he contends, and will be more than offset by the efficiency gains that AI enables. “It’s not like, ‘Oh no, we can’t do it because we’re addicted to doing chat sessions,’” Gates points out, dismissing fears that our growing AI usage might derail climate action.
The race is now on to ensure that breakthrough comes in the form of sustainable innovation rather than increased consumption.

For Pakistan, these developments present both challenge and opportunity. As the country works to modernise its technology sector and harness AI for climate resilience, choices made today will shape the environmental impact for years to come.
Dr Kashif Talpur, a machine learning researcher in UK’s Solent University, emphasises the importance of considering the full lifecycle of AI models. “
“An AI model has to go through two stages: training and inferencing. Inferencing, which occurs when the AI model is in the hands of end users, constitutes about 90pc of its lifecycle. This stage incurs significantly higher costs compared to the prior one, involving millions of dollars, thousands of kilowatts of energy per hour, and substantial carbon emissions. On average, a large AI model generates more carbon footprint in its lifetime than an average American car.”
He further explains the scale of the challenge: “The scale of these models makes them highly energy-demanding, as millions of users submit numerous queries daily, with each query requiring a separate inference. Additionally, speed is a critical efficiency parameter for AI model performance, necessitating powerful, energy-hungry processing units. The more complex the problems these models are expected to solve, the more computational resources each inference requires.”
“Today’s large language models, such as ChatGPT, Gemini, and various other copilots, are equipped with billions of parameters. Maintaining and cooling the infrastructure, like data centres, adds to the ongoing costs in terms of energy consumption, hardware wear and tear, and operational expenses,” Dr Talpur notes.
However, he also sees cause for optimism in technological advancements. “Recent developments in AI hardware, such as specialised computational units like NVIDIA’s AI chips, offer more efficient solutions compared to traditional GPUs and TPUs. These advancements can significantly speed up tasks while reducing energy demands. On the software side, AI and machine learning scientists are developing models with quantisation abilities, which help reduce the computational capacity needed. This shows a promising AI future with environmental issues addressed up to most extent.”
For Pakistan, charting a path forward means investing not just in AI adoption, but in making that adoption sustainable. It means policies that favour renewable energy for potential future data centres, that prioritise water conservation in cooling systems, that incentivise the development of lean, green AI models. It means cultivating local talent and ensuring Pakistani voices are heard in the global dialogue about responsible AI.
Most of all, it means recognising that embracing AI’s transformative potential comes with a responsibility — a duty to deploy these tools in a way that doesn’t exacerbate the very problems they’re meant to solve.
In this respect, Pakistan’s journey with AI is a microcosm of the larger global challenge. As intelligent machines become increasingly essential in the fight against climate change, ensuring they don’t become part of the problem will be a defining test of our time.
The stakes, for Pakistan and the planet, couldn’t be higher. But with foresight and a commitment to sustainability, there’s hope yet that the AI revolution will be a force for healing, rather than harming, our fragile world.
Header illustration created with generative AI
PRISM/DAWN
In a world racing to combat climate change, the newest weapon in our arsenal comes with an ironic twist. Artificial Intelligence, our digital saviour in the fight against global warming, has developed quite a thirst of its own. In what could be a scene from a science fiction story, the very machines we’re building to help save our planet are guzzling water and energy at rates that have scientists very concerned.
Every time you ask ChatGPT — that marvel of modern AI that can write poems about climate change or calculate carbon footprints — a few dozen questions, it drinks roughly 500ml of clean water to cool the powerful computers behind its intelligence. That’s two glasses of water for a few dozen queries, while training a model like GPT-3, which is several generations behind OpenAI’s latest o1 model, consumes millions of litres more.
For Pakistan, perched precariously on the frontlines of the climate crisis, this paradox holds particular significance. In a country where glacial melt threatens northern communities and erratic monsoons flood southern plains, AI offers powerful tools for prediction and adaptation.
Yet as temperature records shatter each summer and water scarcity looms, the environmental cost of these digital solutions cannot be ignored. “Climate change is the biggest challenge facing the planet. It will need every solution possible, including technology like artificial intelligence,” notes Jackie Snow in National Geographic.
But as Sam Altman, OpenAI’s chief executive, recently admitted at the World Economic Forum, AI’s growing energy demands present their own challenge: “There’s no way to get there without a breakthrough.”

At its best, artificial intelligence acts like a planetary nervous system — sensing, predicting, and helping us respond to environmental threats. Google’s Flood Hub, for instance, provides flood forecasts up to seven days in advance across more than 80 countries, helping protect communities across Africa, Europe, South and Central America, and the Asia-Pacific region, including Pakistan. As of 2023, their forecasts cover areas where over 460 million people live. In October 2023, this service expanded to the US and Canada, covering more than 800 riverbanks where over 12m people live.
In California, machine learning algorithms help firefighters predict and track wildfire spread. And in agriculture, AI’s precision farming techniques are reducing water waste and optimising crop yields.
These applications aren’t just impressive — they’re essential. The World Meteorological Organisation estimates that improving early warning systems could reduce climate disaster damages by 30 per cent.
AI is already delivering real climate action results. Global Forest Watch uses AI and satellite imagery to create a real-time tool to monitor and combat deforestation. Google’s AI-powered project Green Light collaborates with 12 cities including Manchester, Rio de Janeiro, Jakarta, and Abu Dhabi to reduce stop-and-start traffic events through AI-supported traffic light management — with early indicators showing potential for up to a 30pc reduction in stops, which could reduce emissions at intersections by up to 10pc. In the fusion energy field, Google DeepMind has developed a deep reinforcement learning system that helps researchers better control nuclear fusion plasma, opening new pathways for clean energy research.
But running these powerful AI systems requires enormous computing power. For example, training GPT-3 consumed an estimated 1,287 MWh (megawatt-hours) of electricity, which is roughly equivalent to the energy consumption of an average Pakistani household over 2,000 years, if the 643 kWh average annual consumption figure is to be believed. This January, the International Energy Agency (IEA) forecast that global data centre electricity demand will more than double from 2022 to 2026, with AI fuelling that increase.
And their carbon footprint is equally large. Training a single large language model is equal to around 300,000kg of carbon dioxide emissions. This is of the order of 125 round-trip flights between New York and Beijing. According to researchers at the University of Massachusetts Amherst, training one of these models can generate carbon emissions equivalent to the lifetime emissions of five average American cars.
The water footprint is equally startling. Modern data centres use vast amounts of water for cooling — up to 500,000 gallons per day for a large facility. By 2027, some experts project that AI systems could demand as much water as half the United Kingdom’s annual consumption.

In west Des Moines, in America’s Iowa state, residents were startled to discover that a nearby data centre — one that helped train some of the world’s most advanced AI models, like GPT-4 — was consuming millions of gallons of their local water supply. A lawsuit by local residents revealed that in July 2022, the month before OpenAI finished training the model, the cluster used about 6pc of the district’s water.
“Within years, large AI systems are likely to need as much energy as entire nations,” warns Crawford. This isn’t hyperbole — the compute power needed for AI training has been doubling every 3.4 months since 2012, a staggering deviation from the traditional 18-month doubling of computer power known as Moore’s Law.
But it’s not just about keeping machines cool. Each component in an AI system’s lifecycle demands resources. Manufacturing the specialised chips that power AI requires ultra-pure water and rare earth minerals. The servers running these systems need constant power, and the batteries storing that power contain materials whose mining creates its own environmental challenges.

While the benefits of AI — improved climate predictions, optimised energy systems, better disaster responses — can be global, the environmental costs fall disproportionately on certain regions and communities.
The disparity is stark: in 2022, while Google operated its data centre in Finland on 97pc carbon-free energy, that number plummeted to between 4-18pc for its data centres in Asia. This dramatic difference means some communities bear a far heavier burden of fossil fuel consumption and air pollution than others. “Unfortunately, there remains a widening disparity in how different regions and communities are affected by AI’s environmental impacts,” note Shaolei Ren and Adam Wierman in the Harvard Business Review.
The scale of this challenge is set to grow dramatically — global AI energy demand is projected to increase tenfold, exceeding the annual electricity consumption of Belgium by 2026. In the United States alone, AI-driven data centre energy consumption is expected to reach about 6pc of the nation’s total electricity usage by that same year.
In water-stressed regions like Pakistan, where every drop counts, the water demands of AI infrastructure could compete with basic needs like agriculture and drinking water.
This disparity extends beyond resources to expertise. According to a Stack Overflow survey published by the OECD, North America hosts 30pc of the world’s AI experts (who are active online), while Pakistan accounts for 0.67pc. This concentration of talent and computing power in the Global North risks creating solutions that may not fully account for the needs and constraints of developing nations.

The path to sustainable AI isn’t just about accepting trade-offs; it’s about reimagining how we build and deploy these systems. Tech companies are making unprecedented investments in renewable energy. According to the latest Solar Means Business report, industry leaders including Amazon, Microsoft, Meta, and Google have signed renewable energy agreements totalling almost 50 GW of clean energy generation capacity — equivalent to the entire power generation capacity of Sweden.
But the real innovations are happening at the intersection of efficiency and design.
“Training better models can actually save more energy over time,” explains Justin Burr from Google AI. “Given the number of times they’re used each day for inference, in less than a week they save more in energy than the old hand-tuned versions.”
Google’s machine-learning optimised Tensor Processing Units (TPUs) demonstrate this principle in action — their version 4 can generate 93pc fewer emissions compared to traditional unoptimised servers.
Location matters too. The carbon intensity of AI training can vary dramatically depending on where the data centres are located. Norway’s electric grid, for instance, produces just 29g of CO2 per kilowatt-hour, compared to 709g in South Africa.
Some regions could turn geography into an advantage. In Quebec, for instance, the combination of hydroelectric power and cold winters creates a unique opportunity: data centres can tap into clean energy while their waste heat is repurposed to warm nearby homes.
“I think that more tax incentives should be given for cloud providers to open data centres in places with hydro or solar energy,” suggests Alexandra Luccioni, an AI researcher quoted in Nature. “In Quebec, we have a very low-carbon grid that relies mostly on hydro, plus with the cold winters, the heat generated by computing centres can be used to heat homes.”
The research community has also been pushing for what they call “Green AI” — a movement that prioritises computational efficiency alongside accuracy. Roy Schwartz and his colleagues found that 90pc of papers at top AI conferences prioritised accuracy over efficiency. But this is changing. Teams like France’s BigScience project have demonstrated that it’s possible to build models similar in size to GPT-3 with a much lower carbon footprint.
Water conservation is becoming a priority too. Meta’s data centres have experimented with raising the temperature and lowering humidity requirements, achieving water savings of up to 40pc in some cases. Google has gone further, pledging to replenish 120pc of the water it consumes by 2030 through initiatives including wetland restoration and rainwater harvesting.
The most promising approaches don’t just focus on individual metrics but take a holistic view of environmental impact. The Copenhagen Centre on Energy Efficiency emphasises that “environmental sustainability should be considered as one of the principles towards responsible development and application of AI”. This means considering everything from the sourcing of materials for AI hardware to the end-of-life disposal of equipment.
“Not every problem demands a machine learning-based solution,” notes Deepika Sandeep, an AI scientist who heads the AI and ML programme at Bharat Light & Power, a clean energy generation company based in Bangalore, India. He advocates for a judicious approach where simpler, less compute-intensive solutions are used when possible.
These improvements can’t come soon enough. But Bill Gates takes a more optimistic view of AI’s environmental equation. While concerns about AI’s energy appetite grow, Gates argues that the technology’s overall impact on climate change will be net positive. The additional strain that AI data centres put on power grids is relatively modest, he contends, and will be more than offset by the efficiency gains that AI enables. “It’s not like, ‘Oh no, we can’t do it because we’re addicted to doing chat sessions,’” Gates points out, dismissing fears that our growing AI usage might derail climate action.
The race is now on to ensure that breakthrough comes in the form of sustainable innovation rather than increased consumption.

For Pakistan, these developments present both challenge and opportunity. As the country works to modernise its technology sector and harness AI for climate resilience, choices made today will shape the environmental impact for years to come.
Dr Kashif Talpur, a machine learning researcher in UK’s Solent University, emphasises the importance of considering the full lifecycle of AI models. “
“An AI model has to go through two stages: training and inferencing. Inferencing, which occurs when the AI model is in the hands of end users, constitutes about 90pc of its lifecycle. This stage incurs significantly higher costs compared to the prior one, involving millions of dollars, thousands of kilowatts of energy per hour, and substantial carbon emissions. On average, a large AI model generates more carbon footprint in its lifetime than an average American car.”
He further explains the scale of the challenge: “The scale of these models makes them highly energy-demanding, as millions of users submit numerous queries daily, with each query requiring a separate inference. Additionally, speed is a critical efficiency parameter for AI model performance, necessitating powerful, energy-hungry processing units. The more complex the problems these models are expected to solve, the more computational resources each inference requires.”
“Today’s large language models, such as ChatGPT, Gemini, and various other copilots, are equipped with billions of parameters. Maintaining and cooling the infrastructure, like data centres, adds to the ongoing costs in terms of energy consumption, hardware wear and tear, and operational expenses,” Dr Talpur notes.
However, he also sees cause for optimism in technological advancements. “Recent developments in AI hardware, such as specialised computational units like NVIDIA’s AI chips, offer more efficient solutions compared to traditional GPUs and TPUs. These advancements can significantly speed up tasks while reducing energy demands. On the software side, AI and machine learning scientists are developing models with quantisation abilities, which help reduce the computational capacity needed. This shows a promising AI future with environmental issues addressed up to most extent.”
For Pakistan, charting a path forward means investing not just in AI adoption, but in making that adoption sustainable. It means policies that favour renewable energy for potential future data centres, that prioritise water conservation in cooling systems, that incentivise the development of lean, green AI models. It means cultivating local talent and ensuring Pakistani voices are heard in the global dialogue about responsible AI.
Most of all, it means recognising that embracing AI’s transformative potential comes with a responsibility — a duty to deploy these tools in a way that doesn’t exacerbate the very problems they’re meant to solve.
In this respect, Pakistan’s journey with AI is a microcosm of the larger global challenge. As intelligent machines become increasingly essential in the fight against climate change, ensuring they don’t become part of the problem will be a defining test of our time.
The stakes, for Pakistan and the planet, couldn’t be higher. But with foresight and a commitment to sustainability, there’s hope yet that the AI revolution will be a force for healing, rather than harming, our fragile world.
Header illustration created with generative AI
No comments:
Post a Comment