Artificial Intelligence Helps Improve NASA’s Eyes on the Sun
A group of researchers is using artificial intelligence techniques to calibrate some of NASA’s images of the Sun, helping improve the data that scientists use for solar research. The new technique was published in the journal Astronomy & Astrophysics on April 13, 2021.
A solar telescope has a tough job. Staring at the Sun takes a harsh toll, with a constant bombardment by a never-ending stream of solar particles and intense sunlight. Over time, the sensitive lenses and sensors of solar telescopes begin to degrade. To ensure the data such instruments send back is still accurate, scientists recalibrate periodically to make sure they understand just how the instrument is changing.
Launched in 2010, NASA’s Solar Dynamics Observatory, or SDO, has provided high-definition images of the Sun for over a decade. Its images have given scientists a detailed look at various solar phenomena that can spark space weather and affect our astronauts and technology on Earth and in space. The Atmospheric Imagery Assembly, or AIA, is one of two imaging instruments on SDO and looks constantly at the Sun, taking images across 10 wavelengths of ultraviolet light every 12 seconds. This creates a wealth of information of the Sun like no other, but – like all Sun-staring instruments – AIA degrades over time, and the data needs to be frequently calibrated.
Since SDO’s launch, scientists have used sounding rockets to calibrate AIA. Sounding rockets are smaller rockets that typically only carry a few instruments and take short flights into space – usually only 15 minutes. Crucially, sounding rockets fly above most of Earth’s atmosphere, allowing instruments on board to to see the ultraviolet wavelengths measured by AIA. These wavelengths of light are absorbed by Earth’s atmosphere and can’t be measured from the ground. To calibrate AIA, they would attach an ultraviolet telescope to a sounding rocket and compare that data to the measurements from AIA. Scientists can then make adjustments to account for any changes in AIA’s data.
There are some drawbacks to the sounding rocket method of calibration. Sounding rockes can only launch so often, but AIA is constantly looking at the Sun. That means there’s downtime where the calibration is slightly off in between each sounding rocket calibration.
“It’s also important for deep space missions, which won’t have the option of sounding rocket calibration,” said Dr. Luiz Dos Santos, a solar physicist at NASA’s Goddard Space Flight Center in Greenbelt, Maryland, and lead author on the paper. “We’re tackling two problems at once.”
Virtual calibration
With these challenges in mind, scientists decided to look at other options to calibrate the instrument, with an eye towards constant calibration. Machine learning, a technique used in artificial intelligence, seemed like a perfect fit.
As the name implies, machine learning requires a computer program, or algorithm, to learn how to perform its task.
First, researchers needed to train a machine learning algorithm to recognize solar structures and how to compare them using AIA data. To do this, they give the algorithm images from sounding rocket calibration flights and tell it the correct amount of calibration they need. After enough of these examples, they give the algorithm similar images and see if it would identify the correct calibration needed. With enough data, the algorithm learns to identify how much calibration is needed for each image.
Because AIA looks at the Sun in multiple wavelengths of light, researchers can also use the algorithm to compare specific structures across the wavelengths and strengthen its assessments.
To start, they would teach the algorithm what a solar flare looked like by showing it solar flares across all of AIA’s wavelengths until it recognized solar flares in all different types of light. Once the program can recognize a solar flare without any degradation, the algorithm can then determine how much degradation is affecting AIA’s current images and how much calibration is needed for each.
“This was the big thing,” Dos Santos said. “Instead of just identifying it on the same wavelength, we’re identifying structures across the wavelengths.”
This means researchers can be more sure of the calibration the algorithm identified. Indeed, when comparing their virtual calibration data to the sounding rocket calibration data, the machine learning program was spot on.
With this new process, researchers are poised to constantly calibrate AIA’s images between calibration rocket flights, improving the accuracy of SDO’s data for researchers.
Machine learning beyond the Sun
Researchers have also been using machine learning to better understand conditions closer to home.
One group of researchers led by Dr. Ryan McGranaghan - Principal Data Scientist and Aerospace Engineer at ASTRA LLC and NASA Goddard Space Flight Center - used machine learning to better understand the connection between Earth’s magnetic field and the ionosphere, the electrically charged part of Earth’s upper atmosphere. By using data science techniques to large volumes of data, they could apply machine learning techniques to develop a newer model that helped them better understand how energized particles from space rain down into Earth’s atmosphere, where they drive space weather.
As machine learning advances, its scientific applications will expand to more and more missions. For the future, this may mean that deep space missions – which travel to places where calibration rocket flights aren’t possible – can still be calibrated and continue giving accurate data, even when getting out to greater and greater distances from Earth or any stars.
Header image caption (same as image in the story): The top row of images show the degradation of AIA’s 304 Angstrom wavelength channel over the years since SDO’s launch. The bottom row of images are corrected for this degradation using a machine learning algorithm. Credits: Luiz Dos Santos/NASA GSFC
NASA Headquarters, Washington
Belgian scientists help develop new method to predict ‘hidden’ solar storms
Sunday, 25 July 2021

An international group of scientists, including from Belgium, have found a new method that can predict stealth coronal mass ejections (CMEs), a type of solar storm, occurring on the Sun’s surface, helping to prevent them from causing damage on Earth.
Most of the Sun is in a charged plasma state, which means the gas is highly ionised, and when such a cloud is ejected by the sun, it is called a plasma cloud or CME.
“Stealth CMEs have always posed a problem, because they often originate at higher altitudes in the Sun’s corona (the outermost part of the Sun’s atmosphere), in regions with weaker magnetic fields,” author Dr Erika Palmerio, a researcher at the Space Sciences Laboratory of the University of California at Berkeley, said in a press release.
“This means that unlike normal CMEs – which typically show up clearly on the Sun as dimmings or brightenings – stealth CMEs are usually only visible on devices called coronagraphs designed to reveal the corona,” she added.
Without spotting CMEs, it is impossible to predict where on the Sun it came from, “so you can’t predict its trajectory and won’t know whether it will hit Earth until it’s too late,” Palmerio said.
Continuous, smaller danger
The last time a CME posed a potential threat to people on Earth was just over nine years ago, in July 2012, when an enormous, diffuse cloud of magnetised plasma, tens of thousands of kilometres wide, was ejected by the sun at a speed of hundreds of kilometres per second.
In this case, the CME just missed the Earth because its origin on the Sun was facing away from our planet at the time, however, if it had hit the Earth, it would have disabled satellites, power grids around the globe would have been knocked out, GPS systems, self-driving cars, and electronics jammed, and railway tracks and pipelines damaged.

The new imaging techniques were applied to remote sensing data of the coronal mass ejection on 8 October 2016. Credit: Palmerio, Nitta, Mulligen et al
The cost of the potential damage of such a large-scale CME has been estimated at between €500 billion and €2.2 trillion in the US alone.
The Earth is impacted by lesser versions of CMEs once every three years, and as the CMEs take around more than one day to reach our planet, the technique could give humans time to prepare for the potential geomagnetic storm.
The scientists, including Marilena Mierla and Andrei Zhukov of the Royal Observatory of Belgium, have said the technique, which will help in taking measures to limit damage to electronics and power grids on Earth from the electromagnetic radiation of such eruptions, can be applied immediately.
ANDY TOMASWICK, UNIVERSE TODAY
25 JULY 2021
Space is full of hazards. The Earth, and its atmosphere, does a great job of shielding us from most of them.
But sometimes those hazards are more powerful than even those protections can withstand, and potentially catastrophic events can result.
Some of the most commonly known potential catastrophic events are solar flares. While normal solar activity can be deflected by the planet's magnetic field, resulting in sometimes spectacular auroras, larger solar flares are a danger to look out for.
So it's worth celebrating a team of researchers from the International Space Science Institute which found a way to better track these potentially dangerous natural events.
Extremely large coronal mass ejections (CMEs) are relatively rare, and when they do happen they normally aren't pointed at Earth.
This was the case in 2012, when a massive solar flare missed Earth but could have knocked out power grids and destroyed satellites on an entire hemisphere of the planet.
Flares as large as the one in 2012 are relatively easy to detect using conventional sensing methods, because of their size but also their positioning.
These sensors can watch for signs of brightening on the Sun's surface that are indicative of a solar flare, or watch the flare itself as it passes out of the sun into the blackness of space.
Unfortunately, the same sensing techniques are not able to detect the most important kind of CMEs – those that are aimed right for us but don't cause any brightening.
These CMEs, which don't produce any telltale signs on the Sun's surface, are known as "stealth" CMEs.
Usually, we only notice these when they actually hit the Earth, and don't have a good indication of where they formed on the Sun. However, the researchers used data collected on four stealth CMEs by NASA's STEREO spacecraft that did in fact track them back to their origins on the Sun.
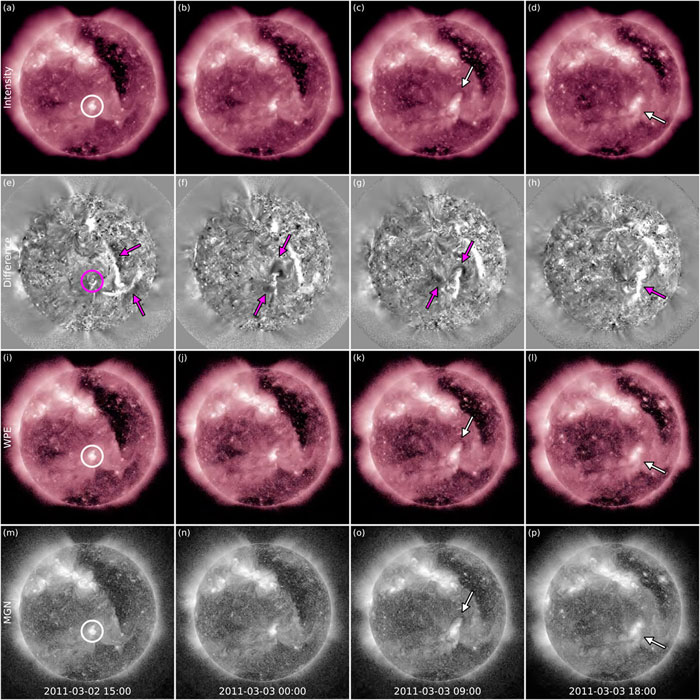
Above: Four different times and imaging techniques capture the 3 March 2011 CME. The top row uses intensity images; the second row uses image differencing with a fixed temporal separation; the third row uses Wavelet Packet Equalization (WPE); and the fourth row uses Multi-scale Gaussian Normalization (MGN). Dimming and brightening regions are indicated with arrows and active region AR 11165 is circled with an arrow in the first column.
When they subsequently analyzed those origin points with other data collected simultaneously, they noticed a changing brightening pattern that appeared for all four stealth CMEs.
They believe these changes are indicative of the stealth CME's formation, allowing scientists precious time to detect and prepare for a potential massive CME hit once similar patterns are detected.
Detecting the patterns themselves can prove tricky though.
STEREO's work in finding the source region of the CMEs used in the study was simply lucky – the spacecraft happened to be looking in the right place at the right time.
To fully flesh out this technique, more data from an angle off-set from Earth will be needed to model the structure of the newly found CME and its origin region.
Help is on the way, though – ESA launched the Solar Orbiter last year, which should be able to collect the necessary data as part of its mission.
It can also help with an even more challenging problem – detecting "super-stealth CMEs", which don't show up on a coronagraph, a standard tool used to detect other types of solar flares.
Understanding is the key to defeating, or at least coping with, this potentially deadly environmental hazard. Now we have a tool to predict more of those hazards, and a path forward to detect even more of them.
This article was originally published by Universe Today. Read the original article.
No comments:
Post a Comment