Model used to evaluate lockdowns was flawed
by Lund University
DECEMBER 28, 2020
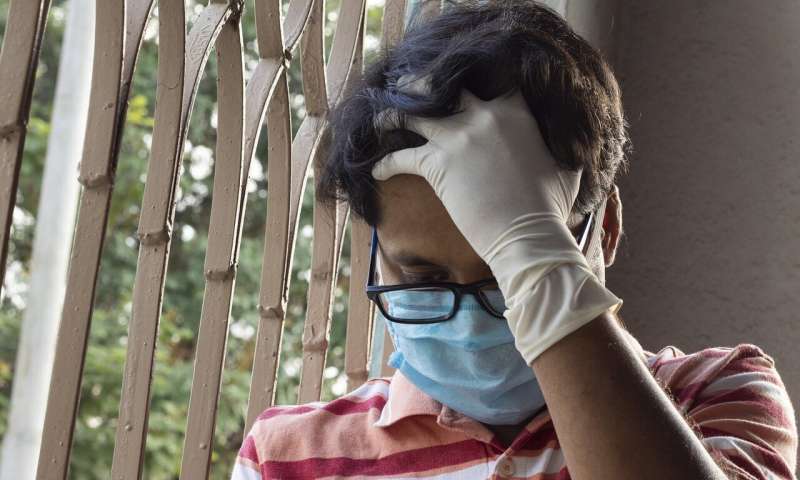
by Lund University
DECEMBER 28, 2020
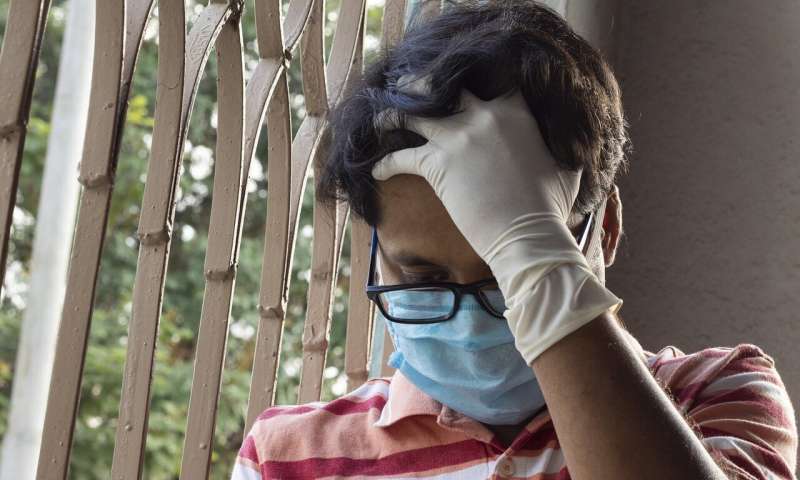
Credit: CC0 Public Domain
In a recent study, researchers from Imperial College London developed a model to assess the effect of different measures used to curb the spread of the coronavirus. However, the model had fundamental shortcomings and cannot be used to draw the published conclusions, claim Swedish researchers from Lund University, and other institutions, in the journal Nature.
The results from Imperial indicated that it was almost exclusively the complete societal lockdown that suppressed the wave of infections in Europe during spring.
The study estimated the effects of different measures such as social distancing, self-isolating, closing schools, banning public events and the lockdown itself.
"As the measures were introduced at roughly the same time over a few weeks in March, the mortality data used simply does not contain enough information to differentiate their individual effects. We have demontrated this by conducting a mathematical analysis. Using this as a basis, we then ran simulations using Imperial College's original code to illustrate how the model's sensitivity leads to unreliable results," explains Kristian Soltesz, associate professor in automatic control at Lund University and first author of the article.
The group's interest in the Imperial College model was roused by the fact that it explained almost all of the reduction in transmission during the spring via lockdowns in ten of the eleven countries modeled. The exception was Sweden, which never introduced a lockdown.
"In Sweden the model offered an entirely different measure as an explanation to the reduction—a measure that appeared almost ineffective in the other countries. It seemed almost too good to be true that an effective lockdown was introduced in every country except one, while another measure appeared to be unusually effective in this country", notes Soltesz.
Soltesz is careful to point out that it is entirely plausible that individual measures had an effect, but that the model could not be used to determine how effective they were.
"The various interventions do not appear to work in isolation from one another, but are often dependent upon each other. A change in behavior as a result of one intervention influences the effect of other interventions. How much and in what way is harder to know, and requires different skills and collaboration", says Anna Jöud, associate professor in epidemiology at Lund University and co-author of the study.
Analyzes of models from Imperial College and others highlight the importance of epidemiological models being reviewed, according to the authors.
"There is a major focus in the debate on sources of data and their reliability, but an almost total lack of systematic review of the sensitivity of different models in terms of parameters and data. This is just as important, especially when governments across the globe are using dynamic models as a basis for decisions", Soltesz and Jöud point out.
The first step is to carry out a correct analysis of the model's sensitivities. If they pose too great a problem then more reliable data is needed, often combined with a less complex model structure.
"With a lot at stake, it is wise to be humble when faced with fundamental limitations. Dynamic models are usable as long as they take into account the uncertainty of the assumptions on which they are based and the data they are led by. If this is not the case, the results are on a par with assumptions or guesses", concludes Soltesz.
Explore further COVID-19 in England: Analysis of the first two waves
In a recent study, researchers from Imperial College London developed a model to assess the effect of different measures used to curb the spread of the coronavirus. However, the model had fundamental shortcomings and cannot be used to draw the published conclusions, claim Swedish researchers from Lund University, and other institutions, in the journal Nature.
The results from Imperial indicated that it was almost exclusively the complete societal lockdown that suppressed the wave of infections in Europe during spring.
The study estimated the effects of different measures such as social distancing, self-isolating, closing schools, banning public events and the lockdown itself.
"As the measures were introduced at roughly the same time over a few weeks in March, the mortality data used simply does not contain enough information to differentiate their individual effects. We have demontrated this by conducting a mathematical analysis. Using this as a basis, we then ran simulations using Imperial College's original code to illustrate how the model's sensitivity leads to unreliable results," explains Kristian Soltesz, associate professor in automatic control at Lund University and first author of the article.
The group's interest in the Imperial College model was roused by the fact that it explained almost all of the reduction in transmission during the spring via lockdowns in ten of the eleven countries modeled. The exception was Sweden, which never introduced a lockdown.
"In Sweden the model offered an entirely different measure as an explanation to the reduction—a measure that appeared almost ineffective in the other countries. It seemed almost too good to be true that an effective lockdown was introduced in every country except one, while another measure appeared to be unusually effective in this country", notes Soltesz.
Soltesz is careful to point out that it is entirely plausible that individual measures had an effect, but that the model could not be used to determine how effective they were.
"The various interventions do not appear to work in isolation from one another, but are often dependent upon each other. A change in behavior as a result of one intervention influences the effect of other interventions. How much and in what way is harder to know, and requires different skills and collaboration", says Anna Jöud, associate professor in epidemiology at Lund University and co-author of the study.
Analyzes of models from Imperial College and others highlight the importance of epidemiological models being reviewed, according to the authors.
"There is a major focus in the debate on sources of data and their reliability, but an almost total lack of systematic review of the sensitivity of different models in terms of parameters and data. This is just as important, especially when governments across the globe are using dynamic models as a basis for decisions", Soltesz and Jöud point out.
The first step is to carry out a correct analysis of the model's sensitivities. If they pose too great a problem then more reliable data is needed, often combined with a less complex model structure.
"With a lot at stake, it is wise to be humble when faced with fundamental limitations. Dynamic models are usable as long as they take into account the uncertainty of the assumptions on which they are based and the data they are led by. If this is not the case, the results are on a par with assumptions or guesses", concludes Soltesz.
Explore further COVID-19 in England: Analysis of the first two waves
More information: Kristian Soltesz et al, The effect of interventions on COVID-19, Nature (2020). DOI: 10.1038/s41586-020-3025-y
Estimating the effects of non-pharmaceutical interventions on COVID-19 in Europe, Nature (2020). DOI: 10.1038/s41586-020-2405-7
Journal information: Nature
No comments:
Post a Comment